Reimagining CPV for a Pharma 4.0™ World
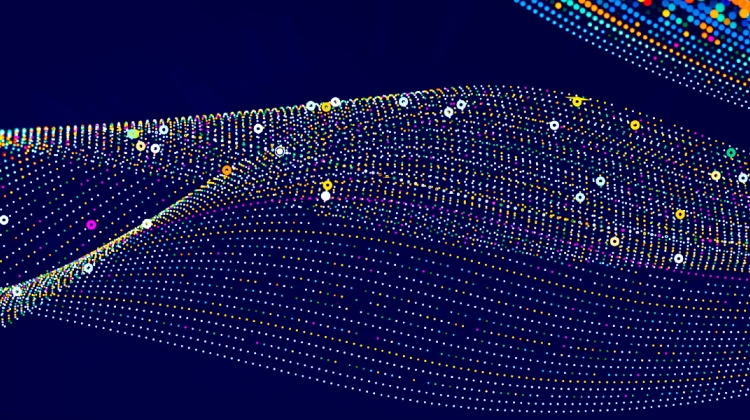
The life cycle approach to process validation stresses the need for continued monitoring of process performance to ensure that the manufacturing process remains stable and predictable, i.e., in a state of control. This life cycle stage is known as continued process verification (CPV) or ongoing process verification (OPV).1 ,2 ,3 In the last decade, regulators have issued revised process validation guidance that puts more emphasis on demonstrating that pharmaceutical manufacturing processes remain in a state of control throughout the product life cycle by applying recurring data analysis.1 ,2
- 1 a b US Food and Drug Administration Center for Drug Evaluation and Research. “Process Validation: General Principles and Practices. Guidance for Industry.” January 2011. ttps://www.fda.gov/regulatory-information/search-fda-guidance-documents/process-validation-general-principles-and-practices
- 2 a b International Council for Harmonisation of Technical Requirements for Pharmaceuticals for Human Use. “ICH Harmonised Tripartite Guideline Q10: Pharmaceutical Quality System.” Published June 2008. https://database.ich.org/sites/default/files/Q10%20Guideline.pdf
- 3International Society for Pharmaceutical Engineering. Good Practice Guide: Practical Implementation of the Lifecycle Approach to Process Validation. North Bethesda, MD: International Society for Pharmaceutical Engineering. 2019.
The process validation life cycle is defined as the collection and evaluation of data from the process design stage throughout production that establishes scientific evidence that a process is capable of consistently delivering quality products.1 It is a regulatory expectation that manufacturers understand their products and processes, regardless of whether products are manufactured internally or at contract manufacturers. This includes periodic review and monitoring to ensure a state of control—see guidance from the US FDA, WHO, and European GMPs, as well as the International Council for Harmonisation of Technical Requirements for Pharmaceuticals for Human Use (ICH) Q8 R2, ICH Q10, and Pharmaceutical Inspection Co-operation Scheme (PIC/S) Annex 15.1 ,2 ,4 , 5 ,6 ,7
The application of CPV/OPV has become a core regulatory requirement and goes beyond traditional annual process reviews (APRs) and product quality reviews (PQRs): CPV programs also monitor critical in-process parameters and material attributes throughout the product life cycle and are typically performed on a more frequent basis. The concepts described in this article should be considered distinct from continuous process verification, which is an alternative approach to traditional process validation in which manufacturing process performance is continuously monitored and evaluated.4 This science- and risk-based real-time approach typically involves inline, online, or at-line controls and monitoring of process performance and product quality on each batch.
However, the combination of Pharma 4.0™ concepts—which enable organizations involved in the product life cycle to leverage the full potential of digitalization to provide faster innovations for patient benefit—and the application of performance-based control strategies may mean these concepts will be one and the same.
Survey of Current Industry CPV Practices
Even though a CPV process has been required by EU GMP regulation since 2015, 5 full implementation is still far from a reality. Completing data gathering, analysis, and reporting at higher frequency than the annual APR/PQR demands resources, which is just one of the many possible reasons for industry's lagging adoption of CPV. To further analyze the factors affecting CPV adoption, we designed and distributed a survey for pharmaceutical companies from countries in the ISPE Iberia Affiliate (Spain and Portugal) to evaluate the level of CPV implementation in those countries.
The survey was completed anonymously by 28 companies. Among them, 75% had 250 or more employees. Although it is not a large overall number, the variety of profiles including innovator, generic, biologics, CMO, medical device, and API, is significant and therefore the results can be considered representative.
The frequency of reviewing data and issuing CPV reports can be controversial. Some companies still do this annually, integrating this review into the PQR. This is increasingly being questioned in inspections and audits, however, because a retrospective study is not the objective of the CPV; rather, the CPV’s goal is to complete a prospective study with the aim of detecting trends and early signs of loss of process control to take preventive actions for these risks. The study found that 46% of companies may not have yet implemented real (non-annual reporting) CPV when considering frequency.
Data acquisition, from both the process and the controls carried out over the process, often consumes many resources, especially when the information is recorded in a paper or hard-copy format and comes from different sources. The time and staff necessary to gather the data decreases with digitalization (electronic records) and with the integration of the various sources of information into repositories. This allows the export of data to the corresponding analysis and reporting tools—a much more agile way that still preserves data integrity.
Digital data management facilitates obtaining information from the process in real time, which is the spirit and ultimate goal of the CPV. The survey revealed that 46% of responding companies have a medium to advanced level of digital data management with integrated electronic data solutions. A follow-up question about the time necessary to gather the data for CPV per product found that 18% would require less than an hour for this task. This is one of the indicators presented by the most advanced companies in digitization. Other respondents would require four hours or more to gather data for CPV. It is also noteworthy that 82% of companies include critical quality attributes (CQAs), critical process parameters (CPPs), and critical material attributes (CMAs) as variables for CPV monitoring and only 18% measure CQAs alone.
Statistical analysis follows data collection. This can be approached with a classic statistical process control with control charts and capability analysis to verify that the process is stable and capable. In some cases, control chart alerts may indicate a risk to product quality that should be addressed. Other data analysis tools can help identify a problem’s root cause. In pharma, multivariate statistics and other advanced analytical tools are beginning to be used due to their effectiveness in analyzing increasingly large and complex data packages. Most companies use spreadsheets or standard statistical packages, but a significant 11% use advanced analytics.
In relation to the personnel who complete these tasks, 71% of responding companies claim to have personnel trained in statistics or data scientists. Regarding the use of the CPV outcomes, companies were asked: Does your company use the CPV information in any way other than GMP compliance? In response, 32% said no and 68% responded yes. Of that 68%, answers on how CPV information was used fell into the following categories:
- Control of variability and process improvement
- Early detection of trends and changes
- Correlations and troubleshooting
- Use as key performance indicators
- Comparisons between different sites and business decision-making
Finally, we asked if the companies had cost estimates for digitizing or automating the ongoing verification process. Twenty-five percent of the companies had performed this exercise and predicted a period of two to five years for implementation and a cost between €300,000 and €1 million.
CPV Maturity Score
By assigning scores to each survey response, we obtained a classification based on the degree of maturity in CPV practice, meaning in the degree of process monitoring and maintenance of the control state. Approximately 20% of responding companies likely are not complying with current GMP requirements; 50% are complying but do not use the full outcomes of the information provided; and nearly 30% have the appropriate systems to use the full potential of the information to improve both the quality and efficiency of their manufacturing plants.
These findings demonstrate how the technological factor (digitization and systems integration) makes a difference by drastically reducing the need to allocate resources (staff and time) to data collection and the subsequent analysis and reporting. These tasks can be automated, which would allow time and staff to be shifted to more valuable activities, such as improvement and decision-making tasks, based on the available information.
Value Beyond GMP Compliance
The benefits of implementing an automated CPV (or CPV 4.0) extend well beyond compliance. Adequate and timely process monitoring ensures robust processes through early detection of drifts in performance before they result in deviations. In our experience, these deviations not only require time and resources to investigate, but can also sometimes lead to batch failures, supply chain problems, and increased production costs.
Robust processes enable pharmaceutical manufacturers to meet supply chain requirements, including introducing new sources of active pharmaceutical ingredients (APIs) or raw materials and transfers to new sites. Further, CPV monitoring enables and enhances cross-site and cross-product learning. To achieve these advantages, a gradual implementation is recommended.
CPV Personnel
Two groups of personnel are involved in completing CPV. The names of these groups may vary across organizations, so here they are referred to as CPV data analysts and manufacturing staff. Data analysts create the CPV report and implement changes from the improvement actions. Technical service, engineering, and quality assurance staff are some examples of CPV data analysts.
Manufacturing staff are the personnel directly involved in the goods treatment process: operators and their supervisors, operations quality, and maintenance. The manufacturing staff benefit from the knowledge acquired from the CPV report for improvement opportunities that is used to create corrective and preventive actions (CAPAs). The knowledge acquired during the product commercial life is considered valuable for the development staff, both as feedback for continuous improvement and as knowledge to be applied to new products.
Completing CPV
Data Collection
The first step is for CPV data analysts to gather the data. Before a full CPV program is deployed, data may come in different formats: from centralized systems with powerful spreadsheet reports, .txt/.csv/.pdf reports with numerical data that each system
creates, manual data taken from batch reports, or even text data from investigations, reports, and other written documentation that must be manually read to extract relevant data.
When a fully automated CPV process is implemented, all data will be gathered automatically in real time. The system will read from all data sources. It could even be a real-time reading of data sources, and the CPV could also take information from human-written reports such as investigations, although these features may be implemented in later stages of the CPV. Even though the technology to gather data automatically is well established in the market, its implementation may still be one of the biggest challenges because it may require a lot of resources (i.e., money, time, and work). This high resource consumption also means that being too ambitious may drive the project to fail. Implementation phases will be covered later in this article.
Data Formatting and Contextualization
The second step is for CPV data analysts to format and contextualize the data so only relevant data is used in the report and it has the correct format for the software that analysts use to be able to process the data (this is usually referred to as data cleansing). As mentioned, data will come from disparate sources, in different formats, and with different contextualization. Data may be structured in well-formatted tables, but also in plain text with missing metadata (data that gives meaning to the numbers), in pictures, or written by people. When the automated CPV is fully installed, the data-gathering process will also automatically perform the data cleansing process previously mentioned. As a result, the CPV data analysts will have a single and well-structured data repository.
Data Analysis
Once all data are clean, CPV data analysts will use statistical and advanced analytical tools to pull information from the data. This information will, at first, be used for compliance needs such as verifying the process capability and the state of control. In later stages of automated CPV, the data will give deeper insights and increase understanding of the process. And, of course, a fully implemented CPV will generate the report automatically.
Automated VS. Manual CPV
So far, the biggest value yet achieved is changing from a manual to an automatic process. The most obvious benefits of automated CPV are gaining resources such as time and staffing and lowering the risk of manual entry error. These also drive higher data integrity levels. But the real benefit is found beyond automating these tasks. If CPV data analysts do not have to spend time gathering data, cleaning data, and writing reports, they can focus on creating better and more comprehensive insights in a continuous manner. Through statistical analysis (either classical or through machine learning and artificial intelligence), it will be possible to determine the influence of each process parameter (either critical, CPP, or not) over each CQA. Reports could also be configured so that the CPV would indicate the future behavior of the process (predictive information).
CPV would provide a better process understanding. Further, it would allow earlier detection of critical-to-quality events, leading to a more efficient root-cause investigation and issue resolution. Root causes can be derived from complex multivariable models, which are able to define the most influential input variables for the event. In one of the last stages of the CPV, the system would even be able to indicate what actions should be taken (prescriptive information). It would be possible for CPV data analysts to create a new set of CPPs based on what is actually influencing quality, or to update the already existing set.
Future models to be included in fully automated CPV will be able to predict a deviation on a CQA based on how each process indicator affects the process. This is possible because statistical algorithms may discover hidden influences, from process events to the process outcome. This way, the process knowledge that the CPV data analysts obtain from the predictive models will enhance the knowledge from classic process engineering sources based on deterministic models (physical, chemical, and/or thermodynamic laws based). There is even more to come. Once advanced CPVs are implemented together with process analytical technologies, then continuous process verification (validation on real-time basis) and even real-time release testing can be considered.
Benefits of Automated CPV
The first benefit is having quality information about the process performance. Using this information, it is possible to create new indicators in the process, as well as discover the importance of others that may already exist but are unrecognized. These process indicators will not only tell if a variable or CQA has moved out of limits (simple information), but also how big the risk is to product quality during a whole process (complex information). It will also be possible to have all the information on a real-time basis due to automation.
An automated CPV will inform earlier about critical events using predictive algorithms. For manufacturing staff, this means that both expected and unexpected events can be handled in advance. Moving from preventive maintenance to predictive maintenance has many benefits. First, changing from a schedule-
based machine stop to an accurate prefailure machine schedule means fewer stop times throughout the year, which allows more machine availability and, in turn, higher production capability. Next, knowing if a machine or a process is about to fail reduces the number of unexpected stops that could drive to a quality issue and, thus, to a product loss.
Predicting events is not the only advantage. As information may become available faster, quality decisions can also be made faster. Some alarms may be created to prompt because of complex indicators that measure changes over many variables, which will allow more processes to move to approval by exception, lowering the workload of quality departments, reducing human error, and reducing production lead time. This will allow the process to be continuously validated on a real-time basis.
Having complete information about the process and equipment will help process engineers modify the plant to create more efficient and robust processes. This, in turn, will lead to using fewer resources such as fewer intake materials, less energy, and less time. CPV not only increases production capabilities, speed, and quality, but it also reduces the cost of the finished goods.
- 1 a b
- 2
- 4 a b International Council for Harmonisation of Technical Requirements for Pharmaceuticals for Human Use. “ICH Harmonised Tripartite Guideline Q8 (R2): Pharmaceutical Development.” Published August 2009. https://database.ich.org/sites/default/files/Q8%28R2%29%20Guideline.pdf
- 5 a b European Commission. “EudraLex, Volume 4: EU Guidelines for Good Manufacturing Practices for Medicinal Products for Human and Veterinary Use. Annex 15: Qualification and Validation.” Published 2015. https://ec.europa.eu/health/sites/health/files/files/eudralex/vol-4/2015-10_annex15.pdf
- 6Pharmaceutical Inspection Convention/Pharmaceutical Inspection Co-Operation Scheme. “Guide to Good Manufacturing Practice For Medicinal Products. Annex 15.” Published April 2015. https://picscheme.org/users_uploads/news_news_documents/ps_inf_11_2015_pics_gmp_revised_annex_15.pdf
- 7World Health Organization. “WHO Expert Committee on Specifications for Pharmaceutical Preparations. 49th Report.” 2015. WHO Technical Report Series. https://apps.who.int/iris/bitstream/handle/10665/176954/9789240693968_eng.pdf
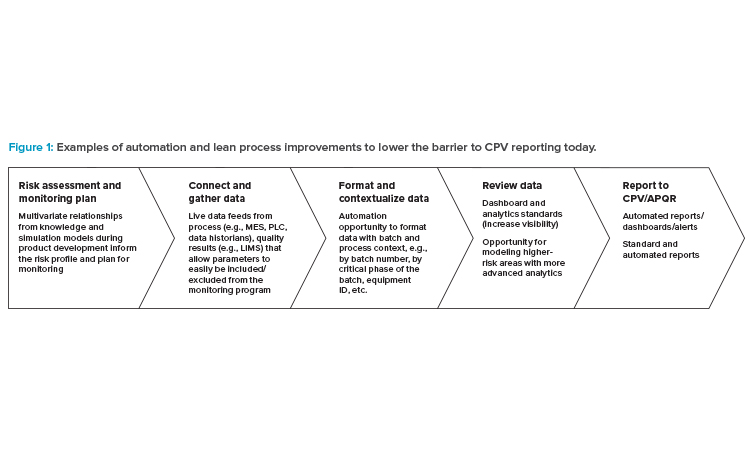
CPV Automation Opportunities
It is evident from the survey results that implementing CPV has not been a straightforward task for many. Digital trends within and outside the industry, specifically advances in data infrastructure and analytics approaches, offer more opportunities to achieve value in process monitoring than a decade ago. Before embarking on any digitalization effort, we recommend identifying opportunities to implement a lean process for CPV (see Figure 1).
Risk-Based Monitoring
Quality risk management is a key enabler for CPV.8 The CPV risk assessment, typically initiated in the development of the product and process, should initially focus on identifying which inputs (typically material attributes, process parameters, analytical methods, manufacturing efficiency, and yield data) could have an impact on the variability of the process output (in process controls [IPCs] or the output CQAs) to determine a monitoring approach that is commensurate with risk.
Consideration must be given to the level of control already in place for a particular input, and whether variability within that level of control is likely to have a significant impact on the CQAs. The experience and knowledge of the cross-functional team should focus on the inputs that are likely to have an actual effect on the variability. Once the inputs and outputs have been collated, the effect of each input must be assessed on the outputs/CQAs. The impact score will be based on the experience and knowledge of the team and, where possible, this will be supported with evidence or prior data. For example, if an input has a specified range (e.g., purchase specification or process control range), but it is known that variation within that range has a significant impact on one or more CQAs or the full range of variation has not been previously observed, then this would score “high” for those CQAs.
Although it is important to trend CPPs from a reporting perspective, they tend to be well controlled with tight acceptance criteria or batch alarms. This means that when modeling these against an output, they do not usually exhibit sufficient variability alone to be strong predictors of an output quality attribute. To truly benefit from multivariate techniques, we need to understand related noncritical parameters, intermediate quality attributes, and other process indicators (e.g., force, power consumption) to determine how difficult it is to control that input process parameter. For example, variations in process indicators can give a clue as to changes within the material being processed. These process indicators, in combination with process parameters and material attributes, can be useful in forming a better picture of what is driving the output variability. It is important that these process indicators are based on robust science from development to factor them into the risk assessment process.
- 8International Council for Harmonisation of Technical Requirements for Pharmaceuticals for Human Use. “ICH Harmonised Tripartite Guideline Q9: Quality Risk Management.” Published November 2005. https://database.ich.org/sites/default/files/Q9%20Guideline.pdf
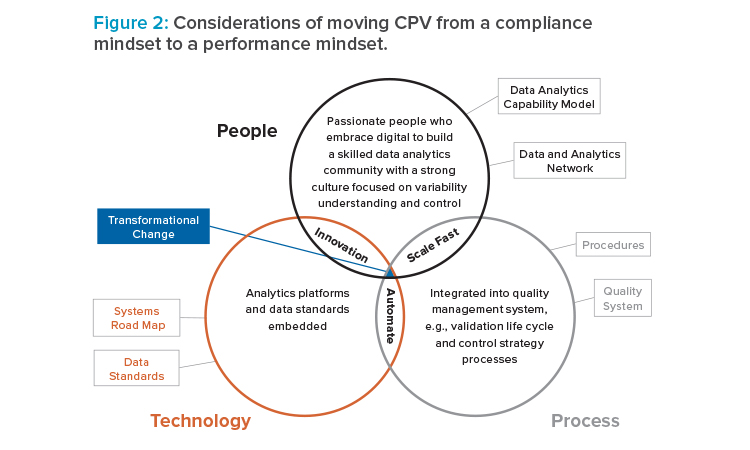
Organization Skill and Cultural Challenges
One of the biggest challenges companies face in the digital transformation are skill and cultural challenges within the enterprise. CPV will lighten the workloads for data analysts, but this also means that they will have to increase their data visualization and data processing skills. A CPV road map must address the need for changing skills and perspectives of personnel (see Figure 2). A transformation process that focuses only on technology is headed to failure.
Figure 3: The CPV 4.0 road map with colors aligned to Gartner’s Types of Analytics Capabilities (2017) terminology.9
- 9Garner Research. “What Types of Analytics and Business Intelligence Are Right for Midsize Enterprises?” Published 14 November 2017. https://www.gartner.com/en/documents/3828063/what-types-of-analytics-and-businessintelligence- are-ri
Steps to Pharma 4.0™ Vision For CPV
For some, Pharma 4.0™ concepts can feel visionary and too future focused. We encourage starting small and anchoring the basis for the CPV data strategy in the risk to the product or patient. While remaining focused on the end vision, we recommend starting with a single product, with the aim to scale an enterprise system for process performance monitoring and control.
Using the road map in Figure 3, we describe some of the processes that need to be in place from pharmaceutical quality system (PQS), culture and skills (C&S), and information technology (IT) system and technology perspectives.
Automate Univariate CQA/CPP Reporting
Using this reporting has many benefits: Compliance with regulatory expectations, an increase in productivity, and the visibility and early detectability of product quality performance. Further, relevant data for reports are easily available for CPV practitioners because the documentation doesn’t require further formatting or adaption. CQA dashboards with signal alerts automate the periodical refresh of this data. Automating the end-to-end process enables GxP reporting for CPV reports and annual product quality review (APQR). Prerequisites for this step include:
- Personnel are skilled in statistics and statistical process control concepts (C&S)
- A continuous quality improvement culture exists (C&S)
- The integrity of the captured data is assured (e.g., systems validated) (PQS)
- Continual improvement of process performance and product quality ICH Q10 (PQS)
- Automated data gathering and contextualization infrastructure is in place (e.g., data lake, historian) (IT)
- All relevant systems integrated provide information to the infrastructure (IT)
Build Models to Understand Multivariate Relationships
This step enables acquiring deeper process knowledge and achieving earlier detection of problems and potential causes. It will create data connections that support adding process parameters and materials attributes for the less-capable CQAs and building genealogies. It will allow offline multivariate models to understand contribution causes of observed variation and multivariate anomaly detection will complement the lagging signal alerting. Prerequisites for this step include:
- Personnel within the CPV team have advanced statistical skills (C&S)
- A data-driven culture exists in the enterprise (C&S)
- A multivariate (statistical) model life cycle management quality procedure is in place (PQS)
- Knowledge management throughout the life cycle ensures science- and risk-based justifications for model relationships (PQS)
- Integration of multivariate statistical software for contribution analysis and batch progression (IT)
Use Online Predictive Models
These models deliver more robust processes, which enhance productivity and reduce cost. Predictive models allow a move to multivariate monitoring to online/real-time analytics (continuous process verification, as briefly described previously). Prerequisite:
- Advanced analytics, simulation, and data science skills in individuals within the CPV team (C&S)
Conclusion
The survey discussed here reveals that manufacturing companies are improving their CPV processes by digitalizing their data-gathering processes and using human teams that have data analysis knowledge. The survey showed that most pharmaceutical manufacturing companies leverage these procedures to gain knowledge and create more robust manufacturing processes. Moving to a fully automated CPV has many benefits, from reducing time to complete CPV to enhancing productivity. For example, automating the first univariate CQA/CPP reports will enhance compliance and online predictive models will enhance productivity and reduce costs.
However, a fully automated CPV is more than a project: it is a journey. Many steps must be completed before a fully automated CPV is up and running, and each step has its own challenges and benefits. To deploy systems capable of gathering data automatically requires an entire IT infrastructure; adaptive process based on automated controls require digital twins and advanced artificial intelligence algorithms; and building multivariate models that will provide a higher process understanding requires a data-driven culture. The road map to CPV shows that the path is not easy or quick, but once implemented, the benefits in productivity and efficiency go far beyond compliance.