Agile, Data-Driven Life Cycle Management for Continuous Manufacturing

Pharmaceutical continuous manufacturing (CM) is recognized as a key process intensification technology, with investment expected to rise in the coming years and the focus shifting toward biologics. This article provides a review on the current state of CM implementation, offers insights into life cycle management and regulatory aspects, and explains how a data- and knowledge-centric approach to risk management can help CM achieve its full potential.
In recent years, global pharmaceutical supply chains, still based on traditional batch- and intermediates-based manufacturing, were overwhelmed by their own vulnerabilities and inefficiencies, and were stressed to the brink of failure as a result of the SARS-COV-2 pandemic. Acknowledging this concern, regulatory agencies have been devising strategies to prepare for future global events.
One approach was to enforce their support for advanced manufacturing approaches such as process intensification by creating working groups such as the Food and Drug Administration’s (FDA) Center for Drug Evaluation and Research’s (CDER) Emerging Technology and Centre for Biologics Evaluation and Research’s (CBER) Advanced Technologies programs, the European Medicines Agency’s (EMA) Process Analytical Technology (PAT) team, and the Pharmaceuticals and Medical Devices Agency’s (PMDA) Innovative Manufacturing Technology working group.1
CM is a method of manufacturing products and processing materials without disruption and with constant material feed and removal. It is recognized as a key process intensification approach. Although it has been widely adopted in other industries, the pharmaceutical industry has yet to do so, and most drug production still relies on the longstanding batch manufacturing process.2 CM can integrate with the PAT and quality by design (QbD) approaches of manufacturing that are capable of increasing the efficiency, flexibility, agility, and robustness of pharmaceutical manufacturing.3 As the pharmaceutical industry shifts its focus to biologics, the investment in CM elements in industry is expected to increase considerably. This is demonstrated by the rise from $1.05 billion US in 2021 to $1.9 billion US in 2022 for the global market share for prescription drugs produced using CM.1 ,4
A Retrospective Journey
In 2015, Vertex’s Orkambi was the first approved drug produced under a CM process for the treatment of cystic fibrosis. Vertex continued to invest in CM with two additional approved drugs—Symdeco/Symkevi in 2018 and Trikafta in 2019—with the same indication. As of 2022, there were 15 drugs (see Table 1) manufactured using CM elements that have received FDA approval, with GSK, Pfizer, and Vertex owning approximately 60% of the market share, followed by Janssen/J&J with about 13%. Several of these drugs, such as Pfizer’s oncology drug Daurismo (glasdegib), were initially approved with a batch production process and then transitioned to CM afterward. AstraZeneca’s Imjudo has some of the isolation steps accomplished continuously.3 ,5 ,6
Historically, the highest number of FDA approvals—60% of total marketed CM drugs—occurred from 2018 to 2020 (see Table 1) and although 2021 approvals decreased to pre-2018 levels, 2022 saw two approvals: 13% of total marketed CM drugs. An average of two approved drugs via CM per year since 2015 shows that progress has been slow but steady and that interest in CM and its potential has been sustained. An integrated end-to-end continuous drug substance to drug product process is yet to be implemented or approved.
Product | Nonproprietary Name | Indication | Company | Approval Year |
---|---|---|---|---|
Orkambi | Lumacaftor, ivacaftor | Cystic fibrosis | Vertex | 2015 |
Prezista1 | Darunavir | HIV | Janssen (J&J) | 2016 |
Verzenio | Abemaciclib | Breast cancer | Eli Lilly | 2017 |
Symdeko/Symkevi | Tezacaftor, ivacaftor | Cystic fibrosis | Vertex | 2018 |
Daurismo | Glasdegib | Myeloid leukemia | Pfizer | 2018 |
Tramacet | Tramadol, paracetamol | Pain | Janssen (J&J) | 2018 |
Trikafta | Elexacaftor, ivacaftor, tezacaftor | Cystic fibrosis | Vertex | 2019 |
Dolutegravir | Doluegravir | HIV | GSK | 2019 |
Fluticasone | Fluticasone ropionate | Allergy relief | GSK | 2019 |
Duvroq | Daprodustat | Renal anemia | GSK | 2020 |
Xofluza | Baloxavir marboxil | Influenza | Roche | 2020 |
Tazverik | Tazemetostat | Follicular lymphoma | Eisai | 2020 |
Cibinqo | Abrocitinib | Atopic dermatitis | Pfizer | 2021 |
Imjudo2 | Tremelimumab | Hepatocellular carcinoma | AstraZeneca | 2022 |
Cibinqo | Abrocitinib | Refractory atopic dermatitis | Pfizer | 2022 |
1 Batch-to-CM conversion
2 Continuous centrifugation and filtration steps
Recent work from the Massachusetts Institute of Technology illustrates its feasibility, with its pharmacy on demand,7 where an end-to-end CM portable refrigerator-sized plant was implemented to manufacture ciprofloxacin. This portable plant consistently achieved drug substance specifications in a fully automated process and with a four-fold increase in the process throughput over its previous iteration. Going up in scale, in a CONTINUUS Pharmaceuticals and MIT collaboration, an end-to-end, QbD-driven integrated continuous manufacturing (ICM) pilot plant8 was designed to produce both small molecule active pharmaceutical ingredient (API) and oral solid dosages. In 2021, CONTINUUS Pharmaceuticals secured a$69.3 million government contract to set up the first FDA-approved end-to-end continuous production facility using its ICM technology.9
Competitiveness Drivers for CM Adoption
Drug manufacturing is still mostly based on the traditional batch approach, for both branded and off-patent manufacturers, through a process of a consecutive number of stepwise unit operations. This entails building a large industrial unit, which requires an investment on the order of billions of dollars for complex equipment and several support systems as well as a large space for controlled storage for raw materials and product intermediates and final product. Additionally, because a large workforce is needed to handle the multiple process and transfer steps, increased risk is introduced for the possibility of contamination and errors.10
Category | Advantages | Description |
---|---|---|
Cost | Reduced floor space and capex/opex costs | A combination of multistep equipment and quality control PAT tools in a single room with dedicated utilities significantly reduces the required capital and operational costs (capex and opex) as well as floor space and product storage needs, with expected savings of about 70%.11 ,12 |
Capacity/Quality/Speed | Real-time quality assurance and improved engineered and automation systems |
A combination of engineering and automation systems with appropriate control strategy enables monitoring and controlling process parameters in real-time, ensuring that critical process parameters (CPPs) remain within the specified design space. This will ultimately improve productivity, efficiency, and the quality of the final product using a QbD approach to manufacturing.10 |
Flexibility/Capacity | Process line flexibility and reduced scale-out/scale-up efforts CM lines can easily be used to promptly develop and produce several new products or as dedicated lines that operate |
CM lines can easily be used to promptly develop and produce several new products or as dedicated lines that operate on an annual basis for a specific product with adjustable throughput depending on demand. The flexibility allows the introduction of extra steps, if needed, and makes process development and scaleup more agile. Furthermore, although scaling up may introduce unwanted risk, a scale-out approach which is well-suited for integration with CM elements may be a beneficial time- and cost-reducing strategy. 13 |
Speed/Cost | Effect on the supply chain | Supply chains for batch-based processes are lengthy and complex and sometimes involve external partners such as contract development and manufacturing organizations (CDMOs). Simplifying the supply chain by reducing storage, negligible intermediates’ shipping costs, and a product delivered in “one-stop shop” fashion will allow more cost-effective decision-making. For example, decisions between the transition from phase II to phase III, which require a significantly increased amount of API material to be manufactured before phase II results become available. Having that capacity flexibility can help avoid investments in unneeded equipment and avoid entrance delays into the market, both of which could result in loss of revenue. |
Operational Flexibility/Capacity | Decentralized manufacturing | Presently, portable end-to-end CM plants are not a widespread reality, but have been proven possible.7 ,8 This decentralized portability with small environmental footprint allows shipping and setup to specific environments and locations, requiring only a few square meters of floor space. Portable CM plants, in turn, can be a response to fast-changing demand trends or specific needs (e.g., local epidemics, military use, space travel). |
Capacity/Quality | More efficient chemistries | CM of small molecules, at times described as flow chemistry, offers numerous benefits over the traditional synthesis path in batch manufacturing. Through flow chemistry, the possibility of more effective and sustainable synthetic routes become feasible, which were previously difficult to scale up in batch, due to lesser heat exchange demands, better contact with catalysts, more optimized exposure to light, more efficient mixing, and ultimately resulting in higher yields and more sustainable processes (see Figure 1). |
Cost | Societal benefits | A positive societal impact is expected with a more ubiquitous presence of CM because it can reduce the cost of drugs and environmental impact, benefiting healthcare and environmental systems, respectively. A broader range of novel dosage forms can be developed for the patients, and even individualized manufacturing, without extensive alterations to the process. |
Speed/Quality/Cost | Artificial intelligence | AI implementation lends itself to the full spectrum of pharmaceutical development, from drug discovery to process design and scaleup to the potential prediction of treatment results.14 This is particularly beneficial for CM, where advanced process control strategies are enabled15 through the integration of machine learning models, creating a superior monitoring framework in the prediction of progression of a process, trend monitoring, and fault detection and therefore reducing development time, process downtime, and waste.16 ,17 |
Speed | Approval times | Analysis of submission data has shown that CM applications are approved three months faster than batch applications and reach marketing four months and one month faster after regulatory submission and approval, respectively, allowing patients earlier access to CM products.18 |
A process using CM offers several advantages for the pharmaceutical industry and patients, as described in Table 2. Considering the acknowledged advantages to CM, coupled with regulatory agencies beckoning, then the question follows: What is holding back the widespread adoption of CM?
Barriers to Widespread Adoption of CM
The pharmaceutical industry has been slower than others in adopting CM. Interest is at an all-time high with slow but steady progress from an industry application standpoint, but traditional batch production remains the industry’s linchpin. Resistance to CM adoption generally falls into one of the following groups.19 ,20
Equipment
The perception of CM equipment as custom made and not as adaptable as batch equipment has been hindering CM adoption. Traditionally, batch equipment provides impressive flexibility, even though it is limited in design and may have heat and mass transfer limitations. Another industrywide concern is the robustness of equipment parts, especially replaceable elements, which are heavily used in single-use technology and CM equipment. Additionally, CM requires an increased number of sensors and integrations with equipment to empower automation (see Figure 1). Also considering the rare shutdown of process lines there is a high demand for robust equipment that can endure consistent manufacturing efforts and displace their batch counterparts.21 ,22
Currently, about 80 companies are developing and supplying a variety of pharmaceutical CM equipment to produce small molecules and biologics.23 Their collaboration with pharma is pivotal to ensure that the many technologies being developed have a standardized and robust design, a cost similar to batch counterparts and, ideally, a plug-and-play functionality.
Regulatory
One major barrier being identified for this lack of global harmonized guidance is regulatory and scientific standards/requirements.1 ,2 The FDA has been prioritizing advanced manufacturing and adding staff to its Emerging Technology platform, but regulation expectations from other markets (e.g., China and Brazil) can add resistance to approvals. Even if regulators agree, the risk of a delay is enough to break a business case.2 ,5 The lack of more CM-specific guidance for the implementation of ICH Q8 to Q12, which is well-understood for batch manufacturing (BM), 5 coupled with the low-risk-taking nature of the pharma market have inhibited the implementation of novel methods of manufacturing.10
Economic
In 2019, the FDA shared data showing that less than 30% of drug ingredients were manufactured in the US because of the labor and cost advantages that offshoring manufacturing to other developing countries offers.9 As such, major investment is warranted for pharmaceutical companies to fully capitalize on the benefits of CM, and they need to make the hard business choice of investing time and money—with added risk—to widen their manufacturing portfolio versus putting money in established technologies that have been profitable.2
In September 2022, President Biden signed an Executive Order launching the National Biotechnology and Biomanufacturing Initiative to incentivize and support the development and implementation of advanced manufacturing technologies.25 These are the type of transforming incentives that pharmaceutical companies need because typically tax and regulatory incentives have been the main drivers affecting cost and time to market. Ireland, Singapore, and Puerto Rico are key pharmaceutical manufacturing hubs where these incentives have had great impact.19
Right Mindset, Risk Perception, and Subjectivity/Bias
Mental inertia and risk perception are often points of resistance when adopting new technologies in the manufacturing process. The perception of increased risk by the pharmaceutical companies is skewed, coming from their lack of experience with CM: This is the area where regulatory agencies need to introduce specialized teams to develop regulatory standards, harmonize them with guidelines abroad, and ease the entry of early adopters.19 ,26 ,27
- 1 a b c Lovells, H., and S. Slater. “FDA Leads Global Work on Continuous Manufacturing Approaches to Up Quality, Supply Chain Resilience — Long-Awaited ICH Q13 Draft Guidelines on CM of Drug Products Released.” JD Supra. Published 24 August 2021. https://www.jdsupra.com/legalnews/fda-leads-global-work-on-continuous-3803791/
- 2 a b c d Pagliarulo, N. “Pharma’s Slow Embrace of Continuous Manufacturing.” BioPharma Dive. Published 24 September 2018. https://www.biopharmadive.com/news/pharmas-slow-embrace-of-continuous-manufacturing/532811/
- 3 a b Van Arnum, P. “FDA Issues Draft Guidance on Continuous Manufacturing.” DCAT Value Chain Insights. Published 28 October 2021. https://www.dcatvci.org/features/fda-issues-draft-guidance-on-continuous-manufacturing-2/
- 4King & Spalding. “Pharma Investment Trend Report: What We’re Watching in 2023.” Published 13 January 2023. https://www.kslaw.com/news-and-insights/pharma-investment-trend-report-what-were-watching-in-2023
- 5 a b c Chen, R., C. Hwang, K. Lee, A. Zydney, and J. F. Kokai-Kun. “Where Do We Stand On Adopting Continuous Manufacturing For Biologics?” Bioprocess Online. Published 5 December 2022. https://www.bioprocessonline.com/doc/where-do-we-stand-on-adopting-continuous-manufacturing-for-biologics-0001
- 6 a b US Food and Drug Administration. Novel Drug Approvals for 2022. Updated 10 January 2023. https://www.fda.gov/drugs/new-drugs-fda-cders-new-molecular-entities-and-new-therapeutic-biological-products/novel-drug-approvals-2022
- 7 a b Capellades, G., C. Neurohr, N. Briggs, K. Rapp, G. Hammersmith, D. Brancazio, B. Derksen, and A. Myerson. “On-Demand Continuous Manufacturing of Ciprofloxacin in Portable Plug-and-Play Factories: Implementation and In Situ Control of Downstream Production.” Organic Process Research and Development 25, no. 7 (July 2021):1534–46. doi:10.1021/acs.oprd.1c00117
- 8 a b Testa, C. J., et al. “Design and Commercialization of an End-to-End Continuous Pharmaceutical Production Process: A Pilot Plant Case Study.” Organic Process Research and Development 24, no. 12 (December 2020):2874–89. doi:10.1021/acs.oprd.0c00383
- 9 a b Kansteiner, F. “End-to-End: Can Pharma Finally Make the Dream of Continuous Manufacturing a Reality?” Fierce Pharma. Published 1 June 2021. https://www.fiercepharma.com/manufacturing/end-to-end-how-pharma-making-dream-continuous-manufacturing-a-reality
- 10 a b c Jhamb, K. “CONTINUOUS MANUFACTURING—Continuous Manufacturing in Pharmaceuticals: Implications for the Generics Market.” Drug Development and Delivery 19 no. 8 (November/December 2019):26–29. https://d2akihtr51eb46.cloudfront.net/wp-content/uploads/2019/11/December-2019-HI-RES1.pdf
- 11 a b Schaber, S. D., D. I. Gerogiorgis, R. Ramachandran, J. M. B. Evans, P. I. Barton, and B. L. Trout. “Economic Analysis of Integrated Continuous and Batch Pharmaceutical Manufacturing: A Case Study.” Industrial and Engineering Chemistry Research 50, no. 17 (2011):10083–92. doi:10.1021/IE2006752
- 12Khinast, J., and M. Bresciani. “Continuous Manufacturing: Definitions and Engineering Principles,” in Continuous Manufacturing of Pharmaceuticals, pp. 1–31. Chichester, UK: John Wiley & Sons, 2017. doi:10.1002/9781119001348.ch1
- 13Sargent, B. “Scale-Out Biomanufacturing – A Paradigm Change to Scale Up.” Cell Culture Dish. Published 24 January 2018. < a href="https://cellculturedish.com/scale-out-biomanufacturing-a-paradigm-change-to-scale-up/">https://cellculturedish.com/scale-out-biomanufacturing-a-paradigm-change-to-scale-up/
- 14Sartorius. “The Trending Role of Artificial Intelligence in the Pharmaceutical Industry.” Published 18 August 2020. https://www.sartorius.com/en/knowledge/science-snippets/the-trending-role-of-artificial-intelligence-in-the-pharmaceutical-industry-599278
- 15Moore, C. M. V., T. P. Garcia, D. B. Hausner, and I. Ben-Anat. “Holistic Control Strategies for Continuous Manufacturing.” Pharmaceutical Engineering 39, no. 3 (May/June 2019):10–21.
- 16Arden, N. S., A. C. Fisher, K. Tyner, L. X. Yu, S. L. Lee, and M. Kopcha. “Industry 4.0 for Pharmaceutical Manufacturing: Preparing for the Smart Factories of the Future.” International Journal of Pharmaceutics 602 (June 2021):120554. doi:10.1016/j.ijpharm.2021.120554
- 17Roggo, Y., M. Jelsch, P. Heger, S. Ensslin, and M. Krumme. “Deep Learning for Continuous Manufacturing of Pharmaceutical Solid Dosage Form.” European Journal of Pharmaceutics and Biopharmaceutics 153 (August 2020):95–105. doi:10.1016/J.EJPB.2020.06.002
- 18A. C. Fisher, et al. “An Audit of Pharmaceutical Continuous Manufacturing Regulatory Submissions and Outcomes in the US.” International Journal of Pharmaceutics 622 (June 2022):121778. doi:10.1016/J.IJPHARM.2022.121778
- 19 a b c Badman, C., et al. “Why We Need Continuous Pharmaceutical Manufacturing and How to Make It Happen.” Journal of Pharmaceutical Sciences 108, no. 11 (November 2019):3521–23. doi:10.1016/J.XPHS.2019.07.016
- 20Wahlich, J. “Review: Continuous Manufacturing of Small Molecule Solid Oral Dosage Forms.” Pharmaceutics 13, no. 8 (August 2021):1311. doi:10.3390/pharmaceutics13081311
- 21Liquidyne. “Continuous Manufacturing Challenges the Pharmaceutical Industry to Improve.” Published 27 July 2021. https://liquidyneusa.com/blogs/news/continuous-manufacturing-challenges-the-pharmaceutical-industry-to-improve
- 22Page. T., H. Dubina, G. Fillipi, R. Guidat, S. Patnaik, P. Poechlauer, P. Shering, M. Guinn, P. Mcdonnell, and C. Johnston. “Equipment and Analytical Companies Meeting Continuous Challenges. May 20–21, 2014 Continuous Manufacturing Symposium.” Journal of Pharmaceutical Sciences 104, no. 3 (December 2014):821–31. doi:10.1002/jps.24282
- 23Anand, S. “Nothing Ventured, Nothing Gain: Bringing to Light the Continuous Manufacturing Equipment Providers.” Published 22 June 2021. https://www.rootsanalysis.com/blog/bringing-to-light-the-continuous-manufacturing-equipment-providers/
- 25AABB. “Biden Announces Biotechnology and Biomanufacturing Initiative, Inaugural ARPA-H Director.” Published 14 September 2022. https://www.aabb.org/news-resources/news/article/2022/09/14/biden-announces-biotechnology-and-biomanufacturing-initiativeinaugural-arpa-h-director
- 26Vanhoorne, V., and C. Vervaet. “Recent Progress in Continuous Manufacturing of Oral Solid Dosage Forms.” International Journal of Pharmaceutics 579 (April 2020):119194. doi:10.1016/j.ijpharm.2020.119194
- 27Hock, S. C., T. K. Siang, and C. L. Wah. “Continuous Manufacturing Versus Batch Manufacturing: Benefits, Opportunities and Challenges for Manufacturers and Regulators.” Generics and Biosimilars Initiative Journal 10, no. 1 (March 2021):44–56. doi:10.5639/gabij.2021.1001.004
![Figure 1: Merck’s kilogram-scale, plug flow LED-powered photoreactor (adapted from [24]).](/sites/default/files/2023-07/0723_PE_JA_Silva_01.jpg)
Introduction of CM in a company should be treated as a complex and formal project whose scope must include every consideration from people dealing with and contributing to the change process. The life science industry has been slower in adopting CM, and shifting organizational mindsets has been claimed to be the most challenging issue that companies need to overcome. The pharmaceutical quality system (PQS) can only evolve when people feel comfortable in their skillset to make the necessary changes needed to incorporate CM.
Quality Culture and Specific Skillset
For companies to comply with regulatory expectations, appropriate training plans and corresponding records must be in place. Despite the regulatory obligation, the full potential of CM can only be achieved with a highly skilled and knowledgeable workforce. Data science and engineering has taken a leading role in processing large amounts of data, advanced process modeling, and machine learning that can transform data into knowledge. On the other hand, production engineers and operations on the shop floor need to deeply understand not only the mechanical aspects of the new technology, but also the wider framework and QbD paradigm that underlies most of CM operations. Mathematical algorithms must be validated, and model life cycle management ensures full reliance for quality decisions that ultimately lead to batch release to market.
The pharmaceutical industry has been slower than others in adopting CM. Interest is at an all-time high with slow but steady progress from an industry application standpoint.
Companies have been engaged with their CM equipment and services providers to develop training programs allowing them to import that knowledge. It is understood that the specialization in CM is multidisciplinary and constitutes a revolutionary change from what already exists. Executive management are actively seeking for return on investment (ROI) and a slower learning curve can be a hard sell as an overall business case for CM. Therefore, when planning their next project, companies need to ensure training costs will be included in the business case.
Generics and Off-Patent Companies
Generics and off-patent pharmaceutical companies—as well as the Association for Accessible Medicines and others—are looking at CM with caution. Two main points of contention are stalling CM adoption in generics production: profit margins for generics companies and ensuring that the implementation of CM does not negatively impact the pharmaceutical market.10
Typically, pharmaceutical generics companies have low profit margins, making manufacturing cost a pivotal point in why CM is lagging in adoption. These costs are further impacted by the current rising prices of raw materials in the aftermath of SARS-COV-2 pandemic and Europe’s geopolitical scenario. Considering the cost associated with transforming existing batch facilities to continuous production facilities, the necessary PAT-related technologies to implement an adequate control strategy, training of highly skilled teams, and lastly the risks and costs associated with new validations and regulatory approvals, help explain why the CM route for branded and generics pharmaceutical companies should be different.
Additionally, it is key for both patients and generics manufacturers that CM-approved drugs can be produced via BM and that CM technology does not prove to be detrimental for the production of a given drug.10
Infrastructure
Economic infrastructure—such as telecommunications, transport, and electricity—and social infrastructure—such as water supply and waste treatment systems—are a paramount prerequisite for industrialization and economic development. In developing countries, it is estimated that energy costs are significantly higher, up to three times more than in comparable developing regions: This is mainly due to relying on expensive backup generators as a primary energy source, which adversely affects profit margins and increases the risk to manufacturing due to the possibility of power outages. Furthermore, weak transportation networks will hinder manufacturers’ ability to capitalize on regional economies of scale. This lagging in infrastructural development poses a serious challenge to CM implementation.28 ,29
THE ICH Q9(R1) and ICH Q13 Loop – Agile Risk and Data-Driven Life Cycle Management
Many pharma and biopharma companies have been operating with processes in batch mode for their entire history. The quality mindset has grown, with a clear definition of the boundaries of a batch. Therefore, they are in a comfortable position in delimiting the scope of an investigation or a genealogy of a product batch. The introduction of CM has shaken the status quo in relation to the most fundamental quality pillars and an era of quality managers that have been leading the paradigm shift.
Quality risk management (QRM) plays a critical role in ensuring the quality, safety, and efficacy of the products, and its importance has been largely recognized by the International Council for Harmonization of Technical Requirements for Pharmaceuticals for Human Use (ICH), which has included it in several guidelines dedicated to the manufacturing and life cycle management of a pharmaceutical product, namely ICH Q8, Pharmaceutical Development,30 and the more recent ICH Q13, Continuous Manufacturing of Drug Substances and Drug Products.31
In both cases, QRM is considered a support for the implementation of such guidelines, acting as the handler of information (of the QRM process itself and risk assessment data of the product) and also as a destination of information coming from the development and manufacturing processes in the format of scientific knowledge that should be available for future risk assessment.
This interdependency between guidelines is a well-established concept, driving the holistic product development approach. But some gaps are still experienced in the pharmaceutical industry when it comes to clearly defining how and when to incorporate the risk assessment principles into the product life cycle, and how and when to feed product/process data back into the initial risk assessment. These gaps, combined with the ever-increasing usage and complexity of technology and data in the manufacturing processes, are creating the need to minimize the subjectivity in QRM so that the decision-making process can be supported by science, data, and knowledge, as well as be robust enough to face any manufacturing process life cycle challenges or regulatory challenges.
A step toward this goal was taken in ICH Q9(R1), Quality Risk Management,32 after the pinpointing of several deficiencies, such as the underlying subjectivity in QRM, supply chain risks, formality of risk assessment, risk-based decisions, and risk review throughout the product life cycle. The ICH Q9(R1) guideline includes new content that should be considered as support for the pharmaceutical industry to start paving the way for the effective adoption of new technologies and digitalization into the QRM process.
Formality in QRM
ICH Q9(R1) Section 5.1 addresses the level of formality that should be incorporated into a risk assessment exercise by bringing concepts such as formality spectrum, stand-alone QRM reports, and cross-functional QRM teams. Depending on the uncertainty, importance, and complexity of the risk-based decision, the organization should be able to move within this continuum range of formality, with the goal of ensuring a robust risk management. The need to drive this process with structured data and standardized tools is critical, when this newly added section clearly states that “risk scores, ratings and assessments should be based on an appropriate use of evidence, science and knowledge.”
Risk-Based Decision-Making
ICH Q9(R1) Section 5.2 addresses the risk-based decision-making process as a consequence of the previously defined level of formality, alongside the levels of effort and documentation. Similar to the management of formality, the process of decision-making should also be seen as a flexible set of available tools and rules to be selected based on the importance, complexity, and uncertainty of the decision. Even though it should be a flexible process, it must be supported by a structured program that ensures “the integrity of the data that are used for risk-based decision-making” to promote the understanding of the variables being assessed and thus reduce the level of uncertainty associated with the decision.
Managing and Minimizing Subjectivity
ICH Q9(R1) Section 5.3 is dedicated to the subjectivity concept that has been part of the ICH Q9 since its initial release. The goal of the revised content is not to eliminate the subjectivity but to ensure that the organizations are aware and recognize its existence in the form of how different stakeholders perceive hazards, harms, and risks. ICH Q9(R1)’s proposal to mitigate the impact of subjectivity is to drive the organizations to “the proper use of QRM tools and maximizing the use of relevant data and sources of knowledge.” Implementing such tools and procedures allows the definition of proper risk scoring scales, standardized risk questions, and the use of existing data to avoid assumptions and biased decisions, increasing the overall robustness of the QRM program.
The Role of QRM in Addressing Product Availability Risks Arising from Quality/Manufacturing Issues
ICH Q9(R1) Section 6.1 introduces a new focus around the concepts of supply availability and reliability, which is a critical prerequisite for a CM process. QRM should be used to prevent and mitigate drug shortages by:
- Supporting the design of process monitoring systems, ideally with real-time data, allowing the detection of deviations from the state of control
- Supporting the design of facilities and equipment “through the use of modern technology, such as digitalization, automation, isolation technology, amongst others,” allowing the introduction of concepts such as predictive maintenance, thus reducing the risks to supply chain
- Supporting the qualification of supply chain partners over the product life cycle by effectively monitoring performance
Regulatory ICH Q9(R1) and ICH Q13 Driver
The ICH Q9 revision poses itself as an enabler of the ICH Q13 implementation. Because CM processes are highly dependent on robust strategies to manage potential variabilities in product availability as well as quality in equipment performance and in overall output production, having a revised ICH Q9(R1) is creating this regulatory driver to push for the efficient implementation of CM.
This regulatory ICH Q9(R1) and ICH Q13 driver should work as a two-way channel so that the much-needed agility and flexibility still supported by a robust structure are included in the industry in the entire product life cycle, without knowledge silos and acting as a retrofitting loop between the initial stages risk assessment and the later development and production stages (see Figure 2).
Leveraging this data-driven risk assessment will facilitate the development of a control strategy for CM by improving the understanding and management of concepts described in ICH Q13 such as:
- Process dynamics, where the flexible management of transient events is crucial to maintain a state of control
- Changes in production outputs, where changes in run times, flow rates, or scale demand a robust justification and assessment of risks to update the initially established control strategy
- Process monitoring and control, where in-process material attribute measurements and data analysis methods should be described and justified in the control strategy
Having a structured digital loop (see Figure 2) between risk assessments at initial and late stages of the product life cycle, supported by the ICH Q9(R1) and ICH Q13 guidelines, will enable a faster reassessment of the designed process (phase 1), accommodating changes or observed process trends during production (phase 3). Process design reassessments will be driven by process insights and data, which are then incorporated in a predefined formal structure (loop back from phase 3 to phase 2 or phase 1). This retrofitting loop enables the expected formal flexibility, a critical concept for an efficient CM process.
- 10 a b
- 28Azolibe, C. B., and J. J. Okonkwo. “Infrastructure Development and Industrial Sector Productivity in Sub-Saharan Africa.” Journal of Economics and Development 22, no. 1 (2021):91–109. doi:10.1108/JED-11-2019-0062
- 29Brönner, M., M., S. Salah, and M. Lienkamp. “Production Challenges in Least Developed Countries.” Challenges 11, no. 1 (January 2020):1. doi:10.3390/challe11010001
- 30International Council for Harmonisation of Technical Requirements for Pharmaceuticals for Human Use. “ICH Harmonised Tripartite Guideline Q8 (R2): Pharmaceutical Development.” Published August 2009. https://database.ich.org/sites/default/files/Q8%28R2%29%20Guideline.pdf
- 31International Council for Harmonisation of Technical Requirements for Pharmaceuticals for Human Use. “ICH Harmonised Tripartite Guideline Q13: Continuous Manufacturing of Drug Substances and Drug Products. Final Concept Paper.” Published 2018. https://database.ich.org/sites/default/files/Q13_EWG_Concept_Paper.pdf
- 32International Council for Harmonisation of Technical Requirements for Pharmaceuticals for Human Use. “ICH Harmonised Guideline Q9(R1): Quality Risk Management.” Adopted January 2023.
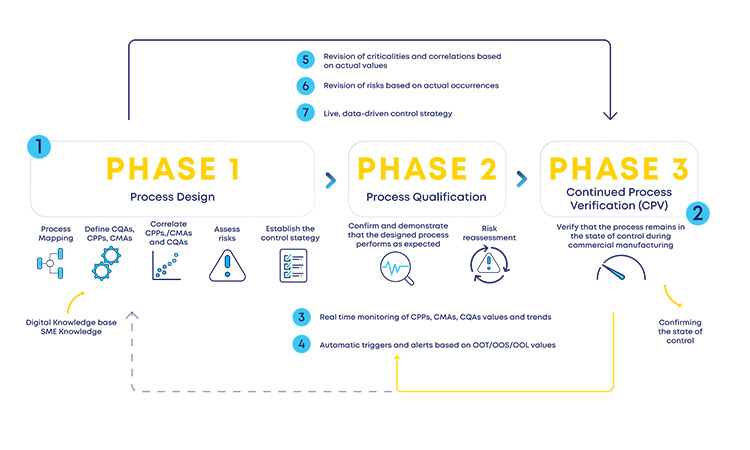
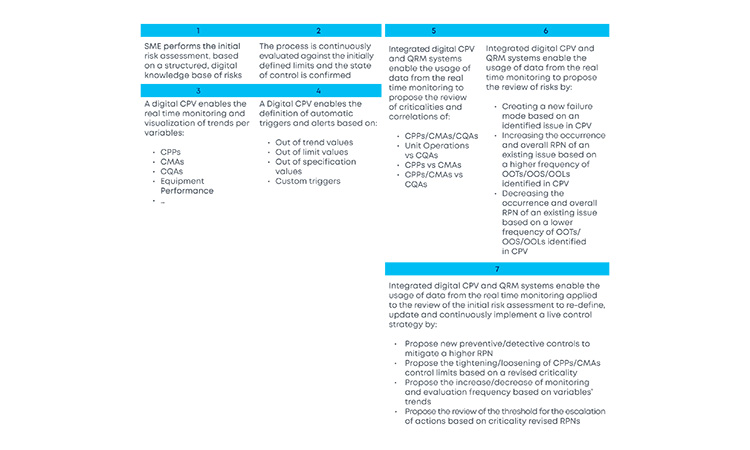
With the new and revised guidance on how to manage data and knowledge about risks and process insights, the proposed loop between the several stages of a product life cycle has gained an increased support in practical application cases. For the sake of simplification, and illustration purposes, the example in Figure 3 covers a single unit operation (continuous filtration).
In a real-life scenario, this would be applied to the whole process. It is shown that, based on a risk assessment performed prior to phase 3, 1 critical process parameters, critical material attributes, and critical quality attributes have been defined for the continuous filtration step. Failure mode effect analysis was used for that purpose, and no critical risks have been identified for this unit operation. As the process enters phase 3, data is continuously gathered and analyzed.3 This data unlocks additional process knowledge which indicates that the defined risk level for the unit operation should be revised based on out of trend (OOT) values that are observed during routine manufacturing.
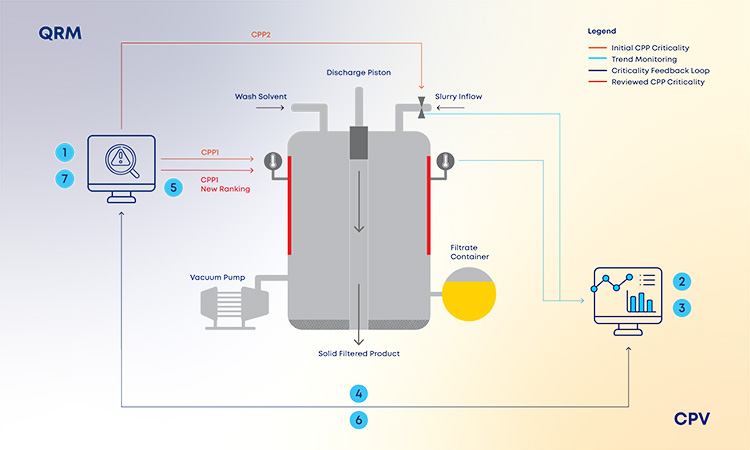
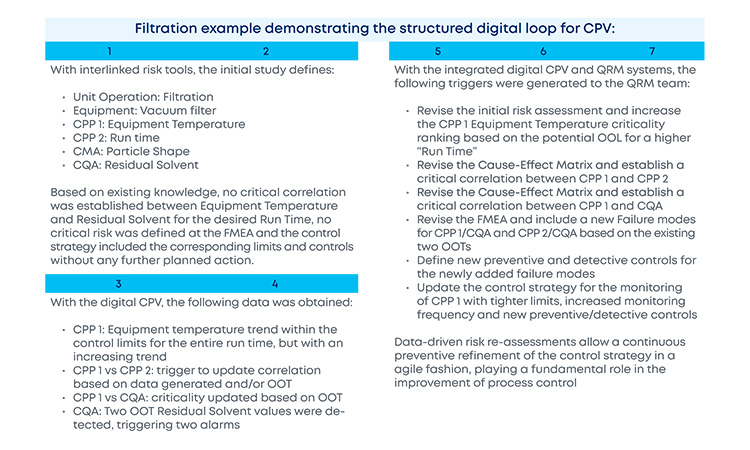
In a manufacturing context, QRM activities and continuous process verification (CPV) are digitally integrated in a retrofitting loop, which dynamically triggers the risk assessment revision,4 with a consequent effect on the refinement of process control strategy.5 ,6 ,7 Risk is thus continuously evaluated based on actual process data and knowledge and process control strategy fine-tuning is performed on a continuous fashion, creating the much-needed process robustness that works as a CM enabler. Additionally, the use of a digitally setup program of QRM and CPV allows data and knowledge to be gathered from the process and then used as support and justification for any proposed process changes, if applicable.
The current industry approach to risk assessment is static in the majority of cases, with risk assessment exercises being executed or revisited at certain defined process life cycle milestones (e.g., prior to PPQ, prior to commercial manufacturing). The value of continuously refining risks based on evidence as part of the ongoing process verification is enormous. The gains are easily picturable: increased process knowledge and increased process control, which consequently reduce OOT and out of specification (OOS) results, financial losses, and compliance risks by approaching the process life cycle with a preventive mindset anchored in robust digital strategies.
Reducing Subjectivity for Quality Decisions
The PQS dictates the ruling principles for quality management within a company where, at a lower level, standard operating procedures and checklists break down those principles into actionable items to batch quality assessment.
The ICH Q9 (R1) guideline opens new horizons in how companies can tackle subjectivity in risk management. Any batch needs to go through a series of quality checks to ensure its safety and efficacy before it can be released for human use. Some of the quality assurance decisions are risk based, highlighting the importance of establishing a systematic approach to risk management where data plays a critical role in increasing process understanding and reducing uncertainty.
CPV and Control Strategy
Far more than conformity against the process range limit or a material specification, a CPV plan, based on sufficient historical data, with a built-in risk to evidence mechanism must be considered as a vehicle to attain such higher levels of quality assurance. Holistic multivariate analysis of the quality attributes of raw material, in-process control, and final product should be a verification point for batch release, including analytical data that goes beyond the formal product specification and that can be a source of valuable insights for verification of state of control.
Subjectivity can also be of concern with material traceability and segregation as well as evaluating the impact of quality planned and unplanned events. Quality decision for automatic segregation of material at risk of nonconformity is governed by automated mechanisms that are driven by instrumentation that acquires and interprets the data using process modeling. The modeling can be validated to ensure not only that the product is within spec when returning to a stationary state, but also how the disturbance affects the operations downstream. Batch genealogy is dynamic because at any point in time a mixture of batches can become components of the final product in proportions that may be estimated. Assessing batch genealogy and material traceability is not deterministic but rather is based on risk, which is refined as more data is generated and process knowledge is increased.
Cleaning Process
A cleaning process is paramount in designing an adequate CM procedure, and an end-to-end risk analysis should be carried out (from input cleaning solutions and sanitizing agents to the analysis of the rinse and swab samples). The traditional way to see cleaning as a “black box”—where what matters is the result of the process—is no longer enough.
Cleaning is a planned event that should occur as infrequently as possible to maximize overall equipment effectiveness. Therefore, the number of batches of product that contacts the equipment surface between two cleaning events is greater when compared to batch mode, even when applying a less conservative non-cleaning program in BM. Risk management workflows and the appropriate tools should be selected to assess the criticality of cleaning process parameters, and cleaning quality assurance should be considered similarly to the manufacturing process.
Having equipment sensors for cleaning process data acquisition and analysis that have the ability to link data to previously identified risks is paramount for a continual risk management cycle and to improve the cleaning process. It will also minimize the risk of a cleaning issue that, in CM, can have dramatic effects in the quantity of product with compromised quality: for example, of an undetected cleaning issue in a previous cleaning cycle.
Quality Investigations
The investigation of quality events in a CM environment brings a new set of challenges when compared to BM due to increased complexity in technology. As such, companies should build their foundations to ensure that batch quality impact assessment and root cause analysis run as smooth as possible. To accomplish this, companies need:
- A multidisciplinary team that is highly skilled with this technology (this may be scarce in some companies)
- A strong QRM foundation, coupled with the availability of data (in the scope of quality investigation, it can support the determination of criticality of the investigation and/or the definition of the scope of the product or potentially impacted systems)
- Digital and subject matter expert (SME) knowledge from similar products manufactured under CM can play a detrimental role, particularly in a context of early adoption
Due to the continuous nature of CM, the number of batches that can be potentially impacted by a quality investigation can be higher when compared with BM. This is the case when a retrospective quality impact assessment needs to be performed with batches that were already quality released.
Conclusion
Despite the current roadblocks to the implementation of CM, there have been clear signs from the different players (e.g., regulatory, equipment manufacturers, academia, governmental entities, among others) in the continued investment in technology. This is expected to rise in the coming years, with the focus shifting toward high-value biologics.
To fulfill all CM potential in process development speed, scalability, and manufacturing throughput, PQSs of pharma and biopharma companies must be restructured on CM’s fundamental pillars in product quality assurance. CM brings a myriad of opportunities, and a clear commitment by the company needs to be made for a product manufactured with this strategy. Companies need to engage in formal change management programs covering people, technology, and processes to support a business case focused on an economically viable ROI for the company.
As mentioned, the current industry approach to risk assessment is static. Typically, risk assessment exercises are executed or revisited at certain de-fined process life cycle milestones. The value of continuously refining risks based on evidence is substantial by approaching the process life cycle with a preventive mindset anchored in robust digital strategies. Leveraging the ICH Q9(R1) and ICH Q13 regulatory package into a structured digital loop that integrates digital process data acquisition (in this article, a CPV example is provided) and QRM systems will drive agile revision of the existing process risk assessment and, potentially, of the control strategy.
Process control strategy refinement will be driven by process insights and data, which are then incorporated in a predefined formal structure. This retrofitting loop between process knowledge and dynamic QRM strategies should be rooted within the company’s PQS, enabling the expected formal flexibility, and reducing subjectivity for quality decisions that, in the end, will guarantee the safety and efficacy of the final product, both critical concepts for an efficient CM process.