Material Properties Databases to Advance Pharmaceutical Processing

Pharmaceutical manufacturers rely heavily on powder processes, the majority of which are designed and operated on the basis of empirical correlations between material properties and performance. The development of material properties databases for pharmaceutical excipients and active pharmaceutical ingredients (APIs) has the potential to enhance such correlations and, more generally, to facilitate activities throughout the pharmaceutical life cycle. A growing body of work in this area shows exciting promise, illustrating the capabilities of material properties databases to add value within the quality by design (QbD) environment that now prevails.
The pharmaceutical industry’s current focus on manufacturing efficiency can be traced back to the early 2000s, when QbD was introduced and generic activity increased. These nearly simultaneous developments resulted in a compelling alignment of regulatory and commercial interests. As the US FDA made clear in 2004,1 “quality and productivity improvement share a common element—reduction in variability through process understanding.” To compete effectively in today’s marketplace, manufacturers require knowledge of how to process materials to a highly consistent product and, by extension, a robust understanding of which material attributes influence in-process behavior. In this context, material properties databases can help advance the science of pharmaceutical processing.
Barriers to Success
Establishing design protocols for powder processes presents a considerable challenge because the behavior a powder exhibits within the process environment is the net result of a complex web of interactions between many variables (see Figure 1). Although chemical properties are critical in powders, as they are in gases and liquids, powder behavior is governed by a wide range of physical variables. Relevant particle properties include size and shape, density, surface roughness, and propensity to accumulate electrostatic charge. Equally influential are system variables such as the amount of entrained gas in the powder, humidity levels, and degree of consolidation.
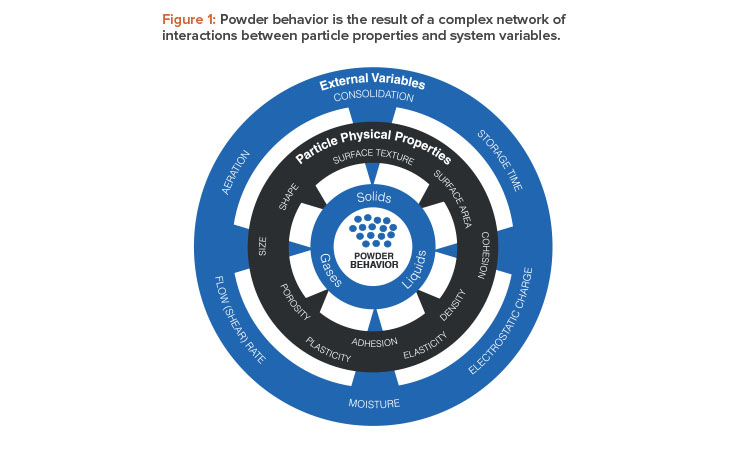
This complexity directly affects powder testing. In particular, manual techniques and techniques that lack a precisely defined methodology or apparatus suffer from poor repeatability and reproducibility, primarily due to the impact of operator-to-operator variability. Because so many variables can impact the outcome of a test, conditions, methods, and apparatus must all be precisely controlled to generate robust data. Furthermore, many powder testing techniques offer minimal facility to control the test environment to produce data relevant to the process of interest—instead, they generate only a single parameter that is meant to reflect the diversity of powder behavior. Relevance and sensitivity are major issues in powder testing. For example, how useful and discriminating are angle of repose data for predicting optimal conditions for a specific formulation in a process as complex as tableting?
Efforts to build a more secure knowledge base for powder processing are crucial but potentially time consuming, and such efforts are carried out against a backdrop of
- intense pressure on research and development’s productivity and time to market,
- significant variability in raw material supply chains, with supplies potentially varying in terms of chemistry (e.g., impurity type and level) and physical properties, and
- complex multistep manufacturing trains, potentially across different locations.
However, significant progress is being made.
Database Benefits
Recently published studies highlight collaborations between academic institutes and major pharmaceutical companies such as GSK, Janssen Pharmaceutica, and AstraZeneca2, 3, 4 to develop extensive material properties databases for pharmaceutical excipients and APIs. Those leading the way in troubleshooting and raw material selection are also increasingly open about the merits of a material properties database–based approach.5 Whether in-house or shared, comprehensive material properties databases for materials in routine use offer considerable potential to:
- Develop robust process models—this is particularly important for the successful adoption of continuous manufacturing, which relies on establishing process control protocols that respond optimally to variability in-process materials.
- Rationalize the number of characterization techniques routinely applied, which is critical for materials in short supply, such as APIs.
- Support the use of surrogates and analogues to overcome limitations with material availability during process development and effectively identify formulation components for generics.
- More effectively set specifications for commercial supplies to more rigorously assess alternatives and secure a robust supply chain.
- Improve process capability metrics by supporting the identification and elimination of potential sources of variability.
These benefits add up to a compelling case for investing in the development of material properties databases.
Data Analysis Strategies
The first step in establishing a material properties database is to consider what can be measured. The number of techniques available for powder characterization studies is now substantial, as evidenced by the extensive study carried out by Van Snick and associates,2 which describes the generation of 100 raw materials descriptors for each of 55 different commercially available APIs and excipients. These descriptors include:
- Particle properties—for example, particle size by laser diffraction, particle morphology by static image analysis, and specific surface area by gas adsorption.
- Bulk powder properties, such as bulk and tapped density, permeability and compressibility, true density by gas pycnometer, moisture sorption and desorption by dynamic vapor sorption, and moisture content by loss on drying. Bulk powder flowability is a focus and was tested via a range of techniques, including shear cell analysis, tapped density, flow through an orifice, angle of repose, avalanche angle, and powder rheometry.
Casting a wide analytical net is essential, but rationalization of the resulting data is key when it comes to the practical application of material properties databases. Multivariate data analysis strategies that have been successfully applied include principal component analysis (PCA) and clustering analysis (CA).2, 3,4
PCA combines individual variables into a limited number of components that capture an acceptable level of variability in the original data set. The application of PCA by Van Snick and colleagues2 identified four distinct components and highlighted flow, cohesion, compressibility, particulate descriptors, permeability, shear descriptors, and water uptake, among other factors, as critical material attributes for the powders tested.
An interesting point to note about PCA is the potential importance of removing multiple highly correlated variables, such as particle size descriptors.2 Laser diffraction produces a range of very highly correlated particle size metrics—including Dv10, Dv50, and Dv90—which can result in an over-weighting of the technique relative to one that provides a single descriptor, such as specific surface area. This point highlights a critical aspect of instrumentation choice. Some systems produce a single parameter, which may or may not be critical; others produce multiple parameters that are highly correlated; and yet others produce multiple parameters that are independently valuable. For example, dynamic, shear, and bulk properties, which quantify many of the critical material attributes listed previously, can all be measured with a single tester (FT4 Powder Rheometer, Freeman Technology). This is an important consideration when comparing the value of different instruments.
CA is a machine learning technique that groups or clusters data points on the basis of similarity or dissimilarity. It can therefore be used to cluster materials with similar properties, as demonstrated by Escotet-Espinoza and coauthors.3 On the basis of CA, a group of 20 materials (with 32 material attributes measured for each) was split into three distinct groups. Flowability and tendency to adhere to processing equipment were identified as key differentiators of the materials in the three groups, with each group then being extremely helpful for identifying surrogates and analogues.
PCA and CA provide valuable insight into the behaviors of pharmaceutical powders, highlighting properties that are correlated or anti-correlated, and the techniques that are most useful. Assessing whether powders with comparable property profiles exhibit equivalent process performance is the crucial final step in confirming the validity of using a material properties database to enhance process-related studies.
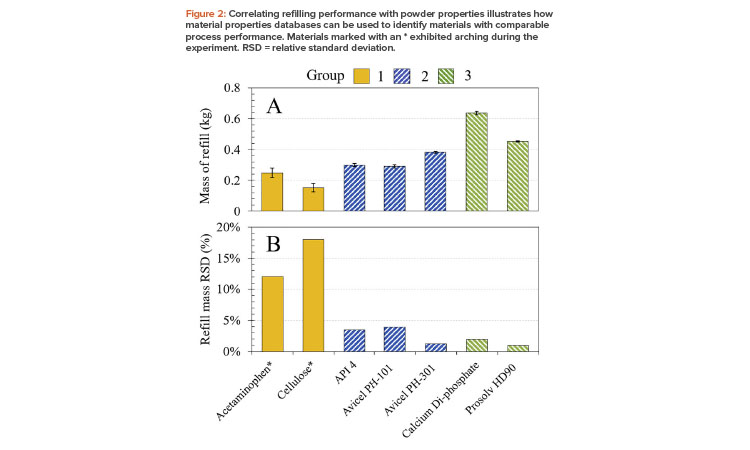
Database Application Example
Figure 2 shows how a selection of seven characterized powders, clustered into the three groups previously described, behave in a feeder refill unit (GEA Process Engineering, Belgium3). This unit consists of an 8-liter hopper located above a rotating cup system; a fixed cup volume of 0.8 liters was used. The mass dispensed into the cup, per actuated refill, was measured for eight refills using a catch scale system (Coperion, K-Tron, Sewell, NJ). Performance was assessed in terms of the mass dispensed per refill, averaged over four refills (Figure 2a), and variability in the mass dispensed per refill (Figure 2b).
The results show that refill mass and the variability associated with dispensed mass are aligned with cluster. Notably, group 1 powders (acetaminophen and cellulose), which have poor flowability, exhibit the most variability in this equipment, dispensing least consistently into the cup. Because these two powders also exhibited arching, it was necessary to hammer the hopper between refills to promote powder flow. More generally, powders lying within the same cluster exhibit similar behavior in the filling operation and indeed in feeder and blender systems, as evidenced by subsequent experiments.
This simple analysis helps illustrate the potential to use material properties databases to develop models for specific unit operations and shows the value of certain measurements for identifying surrogates/analogues. Here, comparability was successfully determined on the basis of dynamic, shear, and bulk properties and particle size data. More specifically, the study highlights the importance of bulk density, permeability, and compressibility in defining process performance, and how dynamic flow energy measurements and shear cell analysis provide independent insights into powder behavior.
Looking Ahead
Powder processes are critical for pharmaceutical manufacturers, and there is considerable merit in collaborative efforts to improve the knowledge base that underpins their application. The establishment of material properties databases is a pragmatic and productive strategy that we have seen success-fully applied by customers across a range of industries.
Over the long term, material properties databases could transform the pharmaceutical industry’s ability to formulate and manufacture with confidence and efficiency. They offer the capability to accelerate the identification of critical material attributes and other aspects of development; develop formulations with specific, predictable physical characteristics; establish appropriately responsive process control protocols; and optimize raw material supply chains. Equally important, material properties databases help differentiate those techniques and instrumentation that offer the most value, supporting the refinement of productive and cost-effective analytical strategies that meet industrial requirements.