Methodology to Define a Pharma 4.0™ Roadmap

In the context of data integrity, data flows are essential. The FDA, PIC/S, and WHO have all emphasized the importance and benefits of data flows in their guidance on data integrity. The key to data integrity compliance is a well-functioning data governance system1, 2 in which the data flow path for all business processes and equipment—such as in manufacturing, laboratory, and clinical studies—is fully understood and documented by a detailed process data flow map.
Based on robust data flows, aspects and required controls can be assessed in detail—for example, manual data entry, interfaces between systems, media change, data conversion, data migration, and data archiving. Process and data flow mapping allow us to apply critical thinking2 to data management and to achieve a holistic approach that not only ensures data integrity, but also offers the most efficient use of data and electronic systems needed for the next step toward digitalization.
This article provides insight into process maps and data flows in the biopharma industry using the Reference Architecture Model Industry 4.0 (RAMI).3, 4 RAMI integrates all assets, including physical items, software, administrative shell, documents, and personnel. It supports the analysis of Industry 4.0 systems and interfaces by mapping them to a three-dimensional (3D) representation. When all assets are integrated, RAMI will enable the transition to full digitalization. The RAMI model is primarily used as a tool for designing integrated operations.
ISPE’s Pharma 4.0™ Process Maps and Critical Thinking Subcommittee offers a perspective on how process data flow maps could be generated using a four-step model. The Subcommittee’s four-step approach starts with mapping existing process. Existing process maps can be used if they are up to date. In this approach, the data life cycle and RAMI are used as tools to map the current state already in the Pharma 4.0™ framework. RAMI is also used later to design the end state. This approach visualizes data and processes from a data-centric perspective, making it easier to identify gaps, inconsistencies, and scope for improvement. By defining an envisioned end state with achievable, intermediate states, an organization will grow and evolve gradually into the new Pharma 4.0™ operating model. It also has a positive impact on maintaining the validated state of the impacted computerized systems and on the planning of budgets and resources. The four-step approach is introduced as a methodology. Each step is explained in the context of the entire process. The concepts introduced here will be topics for future articles.
Pharma 4.0™
One of the principal tenets of Pharma 4.0™ is digitalization, which “will open new horizons to achieve new levels of connectivity, transparency, agility and productivity through the application of faster and more accurate information for decision-making.5 The vision is to design a connected architecture in which data are used as a single source of truth and available at any level at any time. Achieving this vision will require the industry to effectively use all data from and about processes, which can be then actively used for decision-making.
Traditionally the industry used the ISA-95 model for digital systems,6 which put processes at the core. Data were primarily generated at level 1 and 2 systems and then fed through a series of connections up to the business solutions level. However, the process-centric approach of this linear model limited data integrity control capabilities. In an interconnected Pharma 4.0™ world, the hierarchy of systems is moving toward a model where data are connected directly to the data source—the original data—and promote a “single source of truth.”
RAMI 4.0
Industry 4.0 concepts, structure, and methods are being adopted worldwide to modernize manufacturing. Industry 4.0 concepts are being applied to process industries to achieve a holistic integration of automation, business information, and manufacturing execution function to improve several aspects of production and commerce across process industry value chains for greater efficiency. RAMI 4.03 was developed by the German Electrical and Electronic Manufacturers’ Association (ZVEI) to support Industry 4.0 initiatives.
RAMI 4.0 defines a service-oriented architecture (SOA) where application components provide services to the other components through a communication protocol over a network. The goal is to break down complex processes into easy-to-grasp packages, including data privacy and information technology (IT) security. The characteristics of transition from Industry 3.0 to Industry 4.0 can be observed in various aspects, as presented in Table 1.
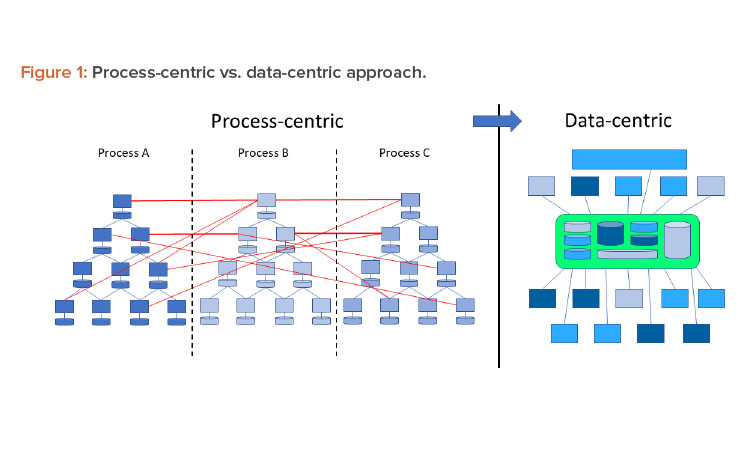
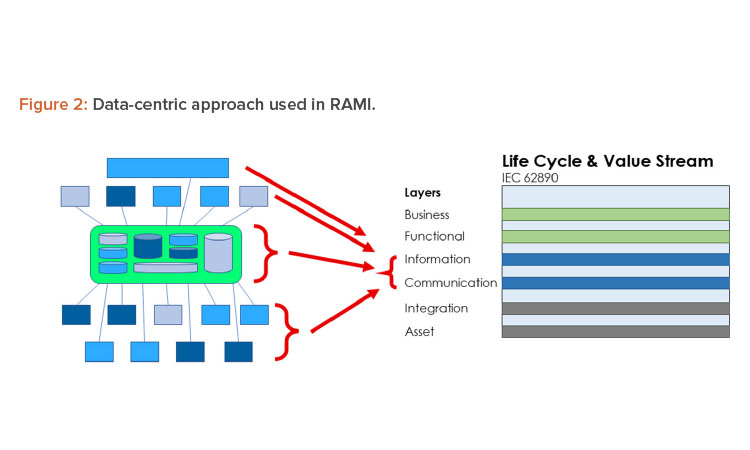
Industry 3.0 Characteristics | Industry 4.0 Characteristics |
---|---|
Hardware-based structure | Flexible systems and machines |
Functions bound to hardware | Functions distributed throughout the network |
Hierarchy-based communication | Participants interact across hierarchy levels |
Isolated product | Communication among all participants |
Product part of the network | |
RAMI 4.0 structure |
Because current processes are not designed based on the Industry 4.0 architecture, this transition will need to be implemented step-by-step. The approach presented next offers guidance for the start of such a transition. The approach can initially be applied to a subprocess, then extended to linked processes. By repeating the approach, the existing processes can be adapted to Industry 4.0 characteristics, resulting in the digitalization needed for the desired levels of connectivity, transparency, agility, and productivity through the application of faster and more accurate information-enabled decision-making.
The Four-Step Approach
In a traditional Pharma 3.0 environment, automation is implemented from a process-centric perspective. This leads to a top-down or vertical automation stack (refer to the ISA-95 model). Data that are generated, collected, and used remain within the boundaries of the process. Where interactions with other processes are required, this is implemented using dedicated and often proprietary interfaces. Due to the lack of a defined structure, these interfaces become very complex and difficult to understand and maintain.
In a Pharma 4.0™ environment, digitalization shifts the focus from the process to the data to achieve new levels of connectivity, transparency, agility, and productivity through the application. Successful digitization requires a data-centric perspective. Therefore, processes should be reviewed from this perspective. Figure 1 shows this shift in perspective.
To implement Pharma 4.0™ to its full extent, a structure is needed to connect and store data in a transparent way and, in turn, should be made available in real time to the target users. The structure designed for this environment is RAMI.4 This model (Figure 2) was initially created to focus on a structured description of a distributed Industry 4.0 system to identify standardization gaps. In this approach, RAMI is used as a tool to design the envisioned end state and to identify optimizations.
To reach the organizational and architectural structure, a basic four-step approach is used that can be continuously repeated to create the level of optimization needed. At its core, the process will document the current state, define the envisioned end state, and construct a path from the current state toward the end state. This path will be split into steps, or work packages, that contain a manageable number of improvements for the organization to take the next step on the path. Figure 3 shows all four steps.
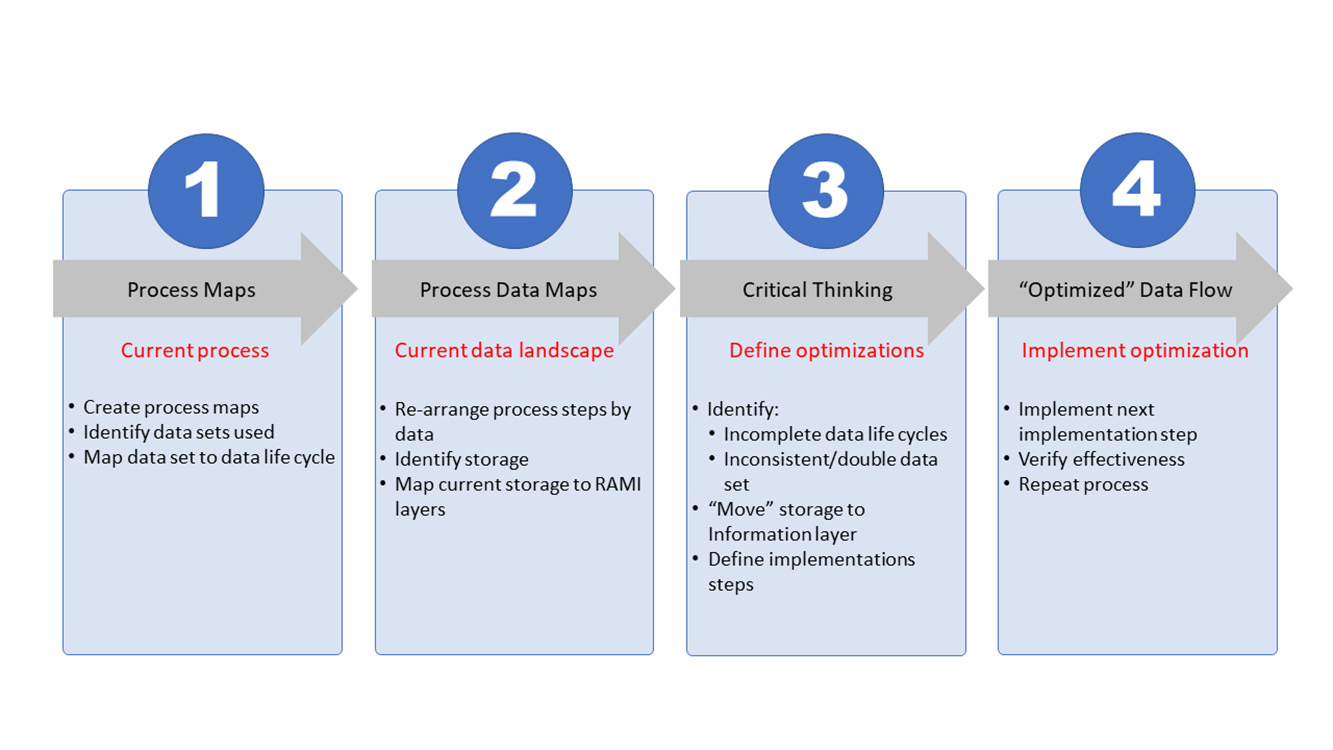

The first two steps (process mapping and process data mapping) are used to document the current state. The third step (critical thinking) is the phase where the envisioned end state is defined and the path to move from current to end state is constructed. In the fourth and last step, the defined work packages are executed. Once a work package is completed, the effectiveness will be assessed where needed.
These four steps are interconnected as the processes provide input for the data mapping. One step leads to the other and the process repeats. Changing the perspective from process-oriented mapping (step 1) to data-oriented mapping (step 2) will provide necessary input for the critical-thinking process (step 3). Process optimization (step 4) is the execution phase and is dependent on the integrated preceding steps. Once process optimization is complete, the effectiveness of the optimization must be assessed, the new current state updated, the envisioned end state verified, and corrective measures, wherever needed, defined. With this, the process becomes iterative toward the envisioned end state and beyond.
In this methodology, the various dimensions of RAMI will be used to analyze the current state (Pharma 3.0 environment) and to determine the desired end state. Analyzing the data life cycle and the RAMI structure regarding the process and data flows gives us insights into the starting point and the interconnectivities.
Process Mapping
The first step toward optimizing production processes is to understand the current state via process mapping. The driver for process mapping in the context of the four-step approach is to lay the foundation for data mapping later. This helps define the origin of the data7, 8 and helps us understand the interdependencies among the disparate processes and systems. It is important to understand that the mapping in this approach is intended to gain insight in one single process, from beginning to end. Therefore, each process will be mapped separately.
In general, process maps provide insight into a process, bring a high-level understanding of each process step, and help identify inefficiencies such as bottlenecks, repetitions, and delays. The process maps also identify inputs and outputs of individual process steps. Many organizations already have documented process maps. These maps provide a good starting point, assuming they are fully up to date.
The core requirement for process-oriented mapping is to have a complete sequential lineup of process steps within a specific process. Figure 4 shows the basis for creating the map. These process steps are recorded in the process lane of the diagram. In this phase, we are also identifying which data sets are used in each process step. This can either be input or output. Therefore, the next step is to identify the data sets as input or output for a process step. Each identified data set will be placed in its appropriate stage of the data life cycle.
A data set is defined as the sum of the data that travels through the data life cycle. The GAMP® structure of the data life cycle is used as a basis, which consists of different phases, namely creation; processing; review, reporting and use; retention and retrieval; and destruction.2 A data set in this context is defined as a conceptual view of a data record, independent of its form.
A data set is only complete when the data life cycle has followed each step and is complete. As discussed later in this article, only complete data sets will be able to transfer to the Pharma 4.0™ environment. However, a data set will almost never complete its full data life cycle within a single process. The result of the first step in the four-step approach is process maps for all the processes. Figure 4 is an example of a simple process.
Process Data Mapping
The process data mapping phase is a detailed exercise to identify the interdependencies on a data level. This means focusing on a single data set and connecting it to process steps in every process to look at the processes from a data point of view. Process data maps link the different processes that interact with the data set and show the data flow.2, 9
To continue process data mapping, a single data set will be mapped. Each process map is reviewed and where the data set is found, the process step is taken and copied into the process data map. Once all process steps are identified and copied, we end up with an overview of all occurrences of the data set. Figure 5 shows all processes of a single data set. Each step that interacts with the data set is copied into the process lane.
Because the data set is only a conceptual representation, we now need to identify where the data set is stored. This can be a reference to a computerized system or a paper document or form. This reference is documented in the storage lane. We will also identify at which level of the RAMI layer a data set currently exists. The following guidance can be used:
- Asset: A data set is not digitized and/or can only be accessed through an asset. Examples include paper forms and documents, files, and databases on a local computer disk not accessible through the network.
- Integration: A data set is digitized, centrally stored, and can be used by multiple processes. However, these processes need to have access to the system managing the data set.
- Communication: Multiple systems can use a data set, usually through dedicated (proprietary) interfaces. Processes can seamlessly use multiple external data sets through a single system.
- Information: Data sets are available to all processes, can be accessed in unified way, and are independent of systems and data formats. This is the targeted layer for Pharma 4.0™ for connectivity, transparency, agility, and productivity.
Note that a data set can go through the various stages of the data life cycle multiple times. Therefore, it may occur multiple times in a data life cycle phase. At this point it is also important to check if a data set touches each life cycle phase, meaning there is a defined process step that creates a data set through a defined process that deletes or destroys the data set. If a step is missing in the life cycle, it should be flagged for further investigation. If a data set is stored at multiple storage points, such as in different databases and/or on paper, it is likely to have redundant data. Data sets should be aligned with regard to data format and metadata to be able to interconnect the data. The data set only completes the data life cycle when the destruction step is reached.
The data set is not yet aligned with the data life cycle phase. Aligning the data set enables us to visualize the current state with regard to storage, duplicates, missing data, or misalignments on data format and metadata. By moving the different process steps to group the data set per life cycle phase, we can visualize where data are created, processed, reviewed, reported, used, retained, retrieved, and, finally, destroyed. Figure 5 demonstrates what an aligned data life cycle for a data set would look like.
Visualizing the process steps through the various data life cycle phases triggers visualization of the disparate data storage and potential redundant data. By following the data life cycle phases in a sequential manner, the potential introduction of any redundant data are visualized and missing data life cycle phases are easily identified. This method of visualization has already inspired us to think about the data architecture and how it interacts with the various processes, which brings us to the next and third step of our methodology—critical thinking.
Critical Thinking
Up this point, the methodology describes the route to visualize the starting situation before beginning the integration to the Pharma 4.0™ architecture. Critical thinking is the core of the approach.
At this stage, the end state will be defined within the architecture of Pharma 4.0™. However, envisioning the end state is not the most challenging aspect. Because the Pharma 4.0™ vision is a paradigm shift from the previous Pharma 3.0, this will not just be a turnkey solution. The most challenging part of the process is uncovering the path to the end state.
For the Pharma 4.0™ environment, it is envisioned to have one single source of truth entering the RAMI architecture. This will affect how processes will be designed. It is not sufficient to create a process within its own silo.
A path needs to take the current position into consideration and move in the direction of the end state. Taking an aerial view, where both the current state and the end state are within scope, will be needed to define intermediate states. Manageable projects or work packages can be created to realize the intermediate states and allow for reflection on achievements: Was the change effective? Does it bring the end state closer? Is correction required? This process is repeated until the envisioned end state has been achieved.
To be viable in a Pharma 4.0™ architecture, data need a set of minimum conditions:
- The data life cycle must be fully implemented.
- Data sets must be free of duplicates (remove redundancies).
- Data sets must be accessible in a unified way.
Using the current state, the end state can be defined considering these three minimum conditions. (The envisioned end state from a data-oriented perspective is shown in red in Figure 5.) To do this, we need to go back to the concept of critical thinking. ISPE GAMP® Guide: Records and Data Integrity defines critical thinking as “a systematic, rational, and disciplined process of evaluating information from a variety of perspectives to yield a balanced and well-reasoned answer.”2 The four-step approach is the systematic process. We have gathered and evaluated our information by creating the process data maps. Now we can analyze them and identify potential issues and opportunities for optimization.
Figure 5 also shows that the data life cycle has been completed by defining the need for a destruction step. But it is not just a matter of defining solutions for the correction and optimization we have identified. In Pharma 3.0, we may have different types of storage within one data set, whereas in Pharma 4.0™, it is envisioned to have one single source of truth entering the RAMI architecture. This is achieved by moving data sets into the information RAMI layer (red dots). To enable this, all the data sets in the “information” layer must be accessible in a unified manner. This means a process must be able to access a required data set in a technology-agnostic way. In principle, a process can access all available data sets within the “information” layer with the appropriate authorization.
The RAMI architecture is designed to seamlessly integrate all layers into a holistic view of the available data. Data creators, such as the assets in the asset layer and the digitized databases in the integration layer, provide their data to convergence information layer. This will be done without needing to know which process is using the data. In the RAMI architecture, the purpose of data creators is to publish. On the other hand, data users, such as functional or business processes, will use the data set without any required knowledge of the underlying assets and databases.
Once the end state is clear, a viable and achievable path must be defined. Because Figure 5 encompasses the entire view for the data set and both the current state and end state are represented, it provides the view we need. A path is created by defining the intermediate stages. An intermediate stage can be defined as the next achievable state that retains the operational state of the organization. A work package or project is a set of activities to realize this intermediate state. Each intermediate result will bring the organization closer to the envisioned end state.
In Figure 5, the identified intermediate states are depicted as green boundaries. Within these boundaries, the change from current state to the new (intermediate) state is shown, with the new state in red. For each state, the activities must be worked out to realize each defined intermediate state. It is to be noted that Figure 5 is merely an example of how the intermediate states can be reached. Because we need to be thinking critically, this approach can change according to the specific needs of a process.
The process lane shows how the different steps, appearing from different processes (Process A, K and Z) are connected in the data set. At this stage we consider the process and the data-oriented mapping to be completed.
- Optimization starts with digitalization of non-digital systems. The first intermediate state, green block 1, shows the transformation from a paper storage system toward the chosen digital storage for the particular data set. In this case the storage system for the data set is a new storage system, i.e., Db Z.
- The next intermediate state, green block 2 shows the existing digital storage system (Db Y) will be transformed to the storage system Db Z.
- Now that the data set is digitalized and transformed into a single storage system, the next step is to integrate this new storage system on the RAMI in-formation layer (green block 3). For example, process step 1 from process A and process step 3 from process K both create data for the data set. Process A creates it in a digitized format at the RAMI integration layer. This data would be available throughout the process. However, process K creates it at the asset layer; in this case on paper. This means that the data are only available within process K if it is executed at the asset itself (the paper). In the previous step, we have already harmonized the data set into a single storage system. By creating the data set in the integration layer, the data set will become available for all processes. The existing processes must be modified to interact with the harmonized data set.
- Data sets are incomplete without a plan for destruction of the data set. Therefore, an integrated method must be designed to destruct the data set at the set destruction time (green block 4).
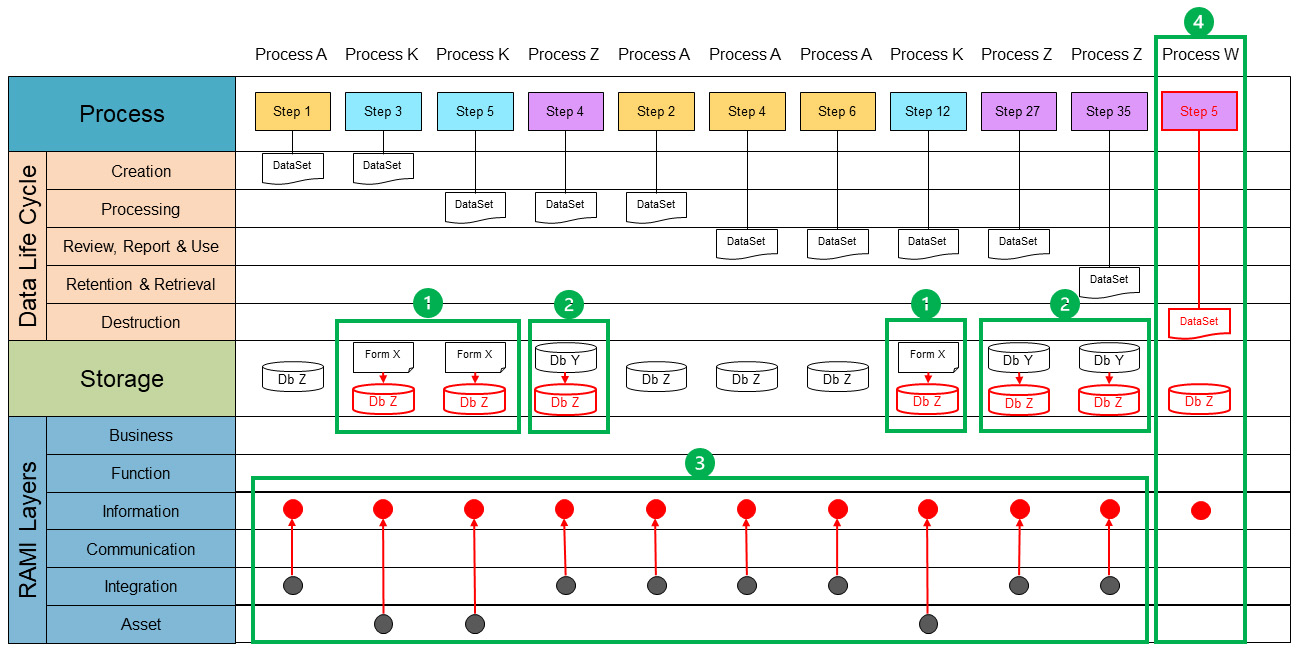
Intermediate step | Description |
---|---|
1 | Transformation from manual storage system to digital storage system for data set (Db Z) |
2 | Transformation from digital storage system Db Y to digital storage system Db Z for data set |
3 | Intermediate actions for integration of the data to be taken for each process step belonging to the data set |
4 | Destruction of the full data set |
Now we have a road map to the envisioned end state and the defined intermediate states that will enable the organization to move toward the end state. The work to realize these steps can now start.
Optimized Data Flows
In this final step, the execution of the work packages starts and the intermediate states will be implemented, bringing us closer to our envisioned end state. The work packages can be handled like any regular project within an organization. This means that not only must funding and resources be secured, but also that the validated state of systems must be maintained. The usual change control and validation activities will be used to govern the implementation of the work packages.
To manage this process, we can use the principles of continuous improvement. With this, the four-step approach becomes an iterative process. Once an intermediate state has been achieved, the following steps will be completed before we can start executing the next work package:
- The current state must be updated. All processes and process data maps must be updated to reflect the new current state. By doing so, we can acknowledge the achievements and ensure we have an up-to-date current state as a basis for further improvement.
- The effectiveness of the implemented work package must be verified. Did it realize the next step toward the envisioned end state? Are all affected processes still in an operational state? Are affected computerized systems still in a validated state? If the goals of the intermediate state have not been fully realized, this will have an impact on the defined path. Where required, any rework or additional work can either be defined as a new intermediate state or be included in the next intermediate state.
- The next intermediate state and envisioned end state must be reviewed. Is the defined end state still valid and viable? Are there new insights and/or new (emerging) technologies that could impact the envisioned end state? Realizing the end state requires mid-to-long-term planning. It is not to be expected that either the organization or technologies remain static.
A review at each intermediate state will help maintain the direction toward the end state, even if this end state changes. These reviews may result in the need for corrective actions or changes in work packages. By using the “critical thinking” principles again, the required actions can be defined and incorporated into the work packages for the next intermediate states. By repeating this process for each of the intermediate states and implementing the required changes in a timely manner, we will ultimately reach our envisioned end state.
We have explained the four-step approach using a simplified single data set. In reality, an organization has many different data sets. Some will be comparable and can be grouped together in work packages and others will require separate work packages. By consistently using the four-step approach, by keeping a holistic view of all business processes and data sets, and by consistently applying the principles of critical thinking, the path to envisioned end state in a Pharma 4.0™ architecture is achievable.
Conclusion
Digitalization opens new horizons to achieve new levels of connectivity, transparency, agility, and productivity by applying faster and more accurate information to automated decision-making. The fundamentals of the digitalization process lie in the structure underneath the data being created, used, reported, stored, and destroyed.
Currently, in the Pharma 3.0 environment, the approach for automation is process-centric. However, moving to Pharma 4.0™, centralizing the data life cycle will lead to a data-centric approach. Each data life cycle needs to be completed and aligned to be considered a data set. In current production processes, different data sets are created for each process. Visualizing the data sets among different process steps will automatically trigger missing, misaligned, or duplicate data. Moving to Pharma 4.0™, each data set will need to be assembled at any time in any business layer. Therefore, a system needs to be in place to show the data architecture and how it interacts with various processes.
The RAMI architecture is originally a design tool, used for designing Pharma 4.0™ processes. In this representation we are not designing new processes; in fact, we are making the transformation from a current situation toward a Pharma 4.0™ architecture. Therefore, the bottom-up RAMI architecture approach, moving from the asset layer toward the business layer, is envisioned.
RAMI is used as a basis to create a data-oriented structure. By analyzing the defined data sets with the RAMI layers in combination with the storage conditions, the gaps for the data-centric approach become clear. During critical thinking, these gaps can be identified as potential issues and opportunities for optimization. Defining intermediate end states with the four-step approach is part of the process in the transformation toward Pharma 4.0™ architecture.
For the Pharma 4.0™ environment, it is envisioned to have one single source of truth entering the RAMI architecture. This will affect how processes will be designed. It is not sufficient to create a process within its own silo. In principle, a process will use data sets that are available within the information layer or create new data sets if not yet available. The process of critical thinking is needed to move forward, to envision the holistic view on data processes, and define intermediate and end states. Existing processes will change in how they need to interact with data.
Once all processes are ready for a single source of truth, the business layer can be looked at in a bidirectional view, where in the second perspective, the business layer defines how the other layers of the RAMI must be integrated to achieve the business objectives. A further goal of the RAMI representation is to create an administrative shell that fully communicates with the integrated systems, databases, applications, etc. The administrative shell contains data storage systems that allow interoperable information exchange via the communication layers. The data are stored in the information layer and then used to exchange data between functions, services, and components.
Using the four-step approach is an iterative process that will lead to the envisioned end state of one single source of truth and using the administrative shell. The key is to start the process small and work consistently and systematically through the different intermediate stages. Only when the four-step approach is complete can another level of complexity can be added, and the four-step approach started again.
To complete the definition of the end state moving toward Pharma 4.0™ production plants, the appropriate technologies to realize this end state must be defined as well. The approach described in this article also raises questions about real-time availability of the data, the speed at which data are available, availability of data to the targeted users, and compatibility among disparate systems. How this can be accomplished—and more detail about the process and data-mapping techniques—is beyond the scope of this article and will be covered by future publications.
Acknowledgments
The authors would like to thank Jonathon Thompson and Menno Broere for their contributions and all the reviewers for providing insights into the article.