Building Better Therapies With Antibody Engineering

Antibody engineering has transformed the development of therapeutic antibodies, enabling the creation of specific and effective treatments for a range of diseases. These antibody-based therapeutics are advancing in clinical development at a rapid rate and are being approved in record numbers. Currently, more than 100 monoclonal antibodies (mAbs) have been approved for the treatment of various disease conditions, including cancers, autoimmune diseases, and chronic inflammatory diseases. However, traditional antibody discovery processes have limitations. Computational approaches have helped researchers overcome those challenges and cleared the way for future discoveries of therapeutic antibodies.
Background on Traditional Antibody Discovery
Traditional antibody discovery processes like phage, yeast, and mammalian display technologies are time-consuming, laborious, and possess several limi-tations, such as identifying the specific antibody binding side (epitope) or obtaining antibodies with optimal properties. Computational approaches have played a critical role in this process, providing researchers with powerful tools to design and optimize antibodies with improved properties. These methods involve the use of advanced algorithms and computational models to predict and model the behavior of antibodies, allowing researchers to optimize key features of the antibody structure.
This article provides a description of therapeutic antibodies, how they are developed, and challenges in the development of antibody-based drugs, and discusses the future roadmap for discovery of therapeutic antibodies.
Antibody: Structure, Functions, and Diversity
Antibodies, also known as immunoglobulin, are protective/disease-fighter protein molecules produced by B cells (specialized immune cells) as a primary immune defense. When pathogens such as bacteria and viruses invade our body, antibodies attach to foreign substances (i.e., antigens) on its surface and help our body to get rid of them. Structurally, antibodies are made up of four polypeptide chains—two heavy (H) chains and two light (L) chains joined to form a Y-shaped molecule (see Figure 1A).
The entire Y-shaped unit can be divided into two main parts: the stem of the Y, known as the fragment crystallizable (Fc) region, and the forks of the Y, known as the fragment antigen-binding (Fab) region. The Fc region is composed of portions of H-chain and L-chain, which have constant amino acid sequences that interact with the receptors present on the cell surface to activate the immune system (i.e., effector function).
The Fab region is composed of the remaining portion of H- and L-chains that are divided into a constant and a variable region. Within the H-chain and the L-chain of the Fab variable region lies a frequently mutated special amino acid sequence region called hypervariable or complementari-ty-determining regions (CDRs) (see Figure 1B). There are six such CDRs. Three of these are present in the L-chain (CDR-L1, CDR-L2, and CDR-L3) and three in the H-chain (CDR-H1, CDR-H2, and CDR-H3). These CDRs are critical for recognizing specific configurations (i.e., epitopes, or antigenic determinants) on the surfaces of antigens and stimulating an immune response.
Moreover, antibody diversity is generated by the combination of variable heavy (VH) and variable light (VL) chains either through variable, diverse, and joining regions (V(D)J), recombination, or somatic hypermutation.1 These two mechanisms together can produce massive antibody diversity (1012–1015) by introducing mutations primarily in the CDR regions. Thus, it increases the probability of recognizing an arbitrary foreign antigen.
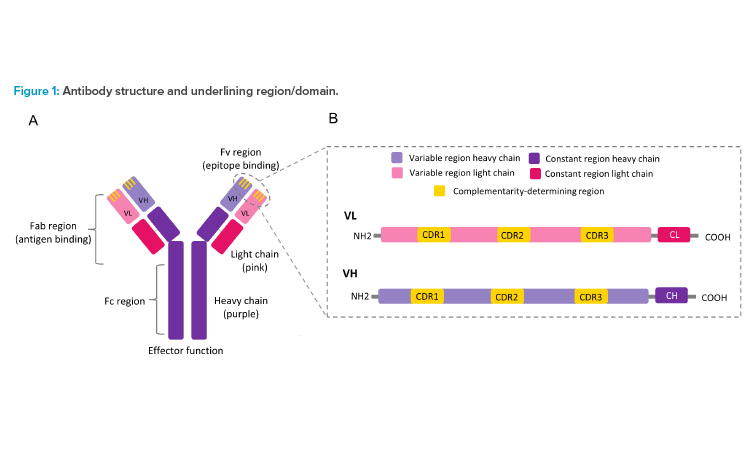
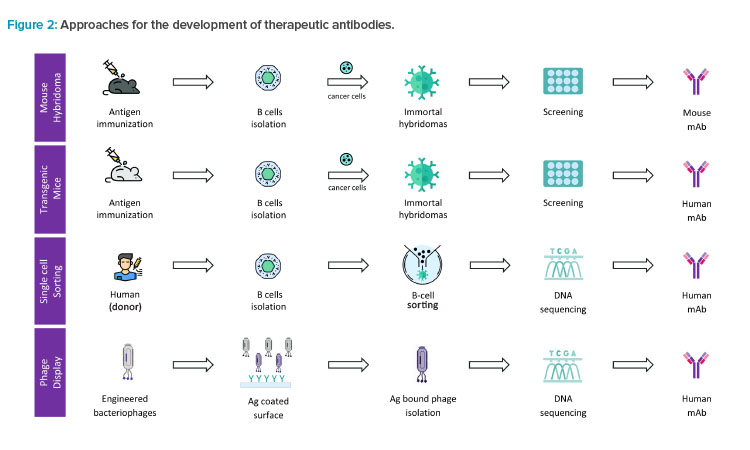
Computational approaches to designing and developing antibody sequence and structure are being increasingly used to complement traditional lab-based processes.
Approaches to Antibody Discovery
Therapeutic antibodies against specific disease targets are developed in a laboratory by mimicking the biological process of the human body and immune system.2 The process begins with the generation of hit molecules by immunizing the host (animals) with the antigen of interest. Some of the methods for hit generation are shown in Figure 2.
Approaches for the development of therapeutic antibodies include the following:
- Mouse hybridoma technique: This method begins by immunizing a mouse with the antigen (Ag) of interest followed by isolation of B cells producing antibodies specific to the antigen. These B cells are fused with cancer cells (myeloma) to produce immortal cells called hybridomas that persistently secrete antibodies.
- Transgenic mice method: In this method, the mice are genetically engineered to produce human antibodies. The mice are immunized with the target molecule and the B cells producing the desired antibodies are isolated and used to produce the antibodies.
- Single cell sorting method: In this technique, B cells are directly sorted from the human (donor) or an immunized animal and screened for the ability to produce antibodies that bind to the antigen molecule. Once a B cell producing a promising antibody is identified, its DNA is sequenced and used to produce the antibodies.
- Phage display method: Also known as surface display method, this technique involves introducing a library of genetically engineered bacteriophages (viruses that infect bacteria) into a sample containing the antigen molecule. The phage that binds to the target is then isolated and the DNA sequence that encodes the binding peptides is determined.
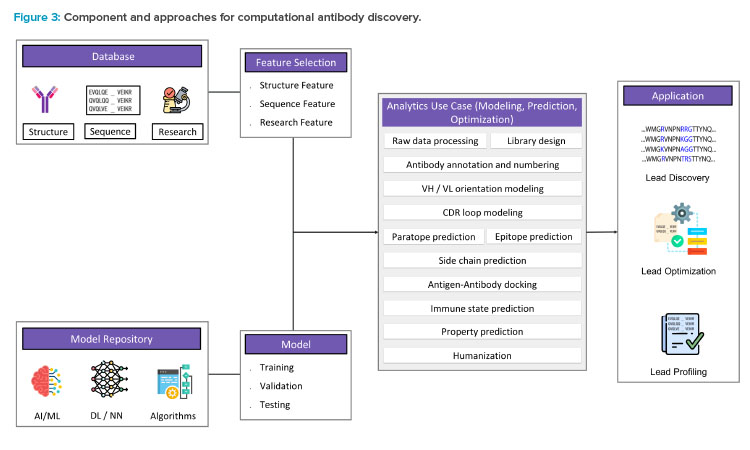
Each method has its own advantages and disadvantages. The method for developing antibodies is selected based on various factors, such as the target molecule, the desired specificity and affinity, and its intended clinical application. In the next phase of the discovery, the emphasis is on optimizing the properties of candidate molecules so that it succeeds in subsequent preclinical and clinical phases. This process is known as lead optimization.
The properties that can be optimized include binding affinity, specificity, selectivity, pharmacokinetics, aggregation propensity, functional activity, and safety. One way of lead optimization is to alter the sequences of the antigen-binding regions (CDR regions) of the antibody to enhance certain properties, such as binding selectivity and affinity.
In this process, VL and VH chains of CDR regions are analyzed, often by next-generation sequencing,3 followed by the creation of variants via mutagenesis or by using synthetic DNA. Through an iterative variant creation and subsequent screening process, lead molecules are selected to move forward in the workflow. These leads are used in in vivo studies to better understand the dynamic interactions with the biological system of interest. This then results in a therapeutic antibody candidate and ends the discovery phase of the drug development process.
Computational Approaches to Antibody Discovery
Computational approaches to designing and developing antibody sequence and structure are being increasingly used to complement traditional lab-based processes. The application of computational biology, artificial intelligence (AI), machine learning (ML), and deep learning (DL) methods to anti-body discovery has rapidly expanded in recent years to address various challenges in the field.4, 5 This includes, but is not limited to, the development of antibody databases,6 molecular modeling and simulations,7 and the use of advanced analytics (see Figure 3).
Homology modeling, molecular docking, and molecular dynamics simulations are commonly used computational biology approaches to predict the 3D structure of an antibody or antigen, and predict antigen-antibody binding affinity and stability.8 Apart from these approaches, various AI, ML, and DL methods have been implemented for prediction and optimization of antibodies. ML and DL algorithms can be trained on the large data sets of protein sequences, structures, and functions to identify patterns and predict outcomes, which is crucial for the development of effective antibodies. Some applications of DL models, such as convolutional neural networks and generative adversarial networks, predict antibody binders and generate synthetic antibodies2 with desired properties and high binding affinity.9
One recent breakthrough was the development of AlphaFold by the Google company DeepMind. AlphaFold is provided as an open access tool to the scientific community. It is a DL-based prediction tool that can accurately predict protein structure based on multiple sequence alignments.10
Because the method relies on sequence alignments for accurate predictions, AlphaFold has limited usefulness in predicting the structure of “orphan” proteins, which have limited sequence information. This limitation is overcome by the development of large language models (LLMs). One example of an LLM is the protein language models, which are trained on large datasets of protein sequences and structures. Recently, Meta launched a breakthrough model called evolutionary scale modeling (ESM). The ESMFold protein language model harnesses the ESM-2 to learn sufficient information to enable accurate, atomic-level predictions of protein structure directly from the individual sequence of a protein.11
ProGen and IgLM are other examples of language models that can generate novel antibody sequences when conditioned on specific inputs. ProGen is a pre-trained language model that can be fine-tuned to predict the binding affinity between antibodies and their targets,12 whereas IgLM is trained to generate synthetic libraries of variable length antibody sequences.13
Key Challenges and a Way Forward
Despite the impressive progress in technologies applied to antibody engineering, developing antibodies with high specificity and binding affinity remain the challenges that require attention. In the future, it may be useful to include codon optimization to finalize antibody designs. Codon optimization is the process of modifying nucleotide sequence of genes based on various criteria without altering the amino acid sequence. Optimization of codon would result in an improvement in protein expression, as well as designing antibodies with high specificity, binding affinity, and biological activity.
Another important outstanding challenge is the de novo loop modeling of antibody CDRs—in particular, the CDR-H3. This is partly due to the large number of degrees of freedom that need to be sampled. Although there is an advance in DL models for protein modeling (AlphaFold2, OmegaFold, ESM-Fold, and Yang-Server) and antibody modeling (IgFold, and NanoNet), modeling of CDR3 is still a big challenge.14 As both quantum computing and AI models advance, optimizing the CDR structure and predicting amino acid substitution would be possible.
The introduction of sophisticated research technologies such as next-generation sequencing (NGS) has significantly increased the speed and breadth of research processes for antibody discovery. NGS provides comprehensive sequence data, enabling the identification, optimization, and characterization of antibodies with desired properties. This has exponentially increased the data volume, which requires high-compute and high-storage solutions. As projects become collaborative in nature, it will become increasingly critical to safeguard the integrity of data security and continuity of operations.
The prospective solution for this would be to establish robust decentralized ecosystems based on the principles of trusted research environments (TREs) that enable data to be jointly analyzed without sharing all aspects of that data. Nevertheless, TREs would also provide a secure and controlled environment without compromising confidentiality.15 This can allow researchers to work together more effectively, accelerate the pace of research, and ultimately lead to the discovery of new antibodies.
Conclusion
The use of computational approaches to design antibodies is increasing and complementing traditional lab-based processes. ML, through embedding and generative models trained on large datasets, represents a new paradigm shift in this field. Biopharmaceutical companies that can generate data at scale will have a significant advantage over others, leading to a focus on digital transformation efforts within the industry. This will include data storage, sharing, and searching, as well as deriving meaningful insights by linking various datasets. Ultimately, the successful translation of AI-enabled antibodies from bench to bedside in record time and at a reduced cost will be the most accurate measure of success.