Continuous Manufacturing as a Tool for Accelerated Development
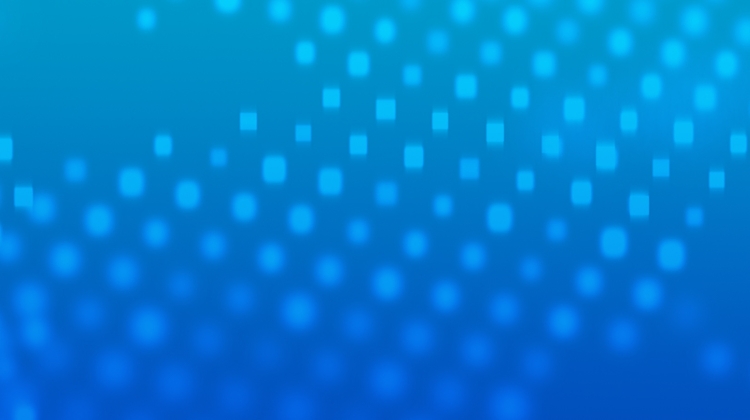
Continuous manufacturing (CM) offers one way the pharmaceutical industry can accelerate development of the drug product control strategy to ensure a robust and reliable supply of medicine to the clinic and/or market. This article explores the promise of continuous manufacturing in enhancing accelerated development, as evidenced by experience in solid oral products.
It has been inspiring to see what our industry has accomplished in an unprecedented time frame in response to the COVID-19 pandemic: development and emergency use authorization for vaccines, neutralizing antibodies, and expansion of previously approved therapeutics for the treatment of COVID-19, to name a few. The industry’s ability to respond to the challenges that the pandemic presented demonstrates the significant value and impact that pharmaceuticals bring to the world, but it also raises the question: How can we apply learnings from our industry’s response to the pandemic to routine development activities to accelerate delivery of medicines to the patients we serve?
The answer to this question is multifaceted, and it will require each function within an organization to assess what role it can play to deliver the solution. As pharmaceutical scientists and engineers, one of our primary responsibilities is developing the drug product control strategy to ensure a robust and reliable supply of medicine to the clinic and/or market. There are myriad ways to accelerate development of the control strategy, but one of the more promising technologies that can facilitate acceleration is continuous manufacturing.
Continuous Manufacturing On the Rise
Since the first US FDA approval of a continuous manufacturing application in 2015, there has been a steady increase in CM’s implementation across the industry. In fact, the 2019 and 2020 Annual Reports from the Center for Drug Evaluation and Research, Office of Pharmaceutical Quality, indicate that at least seven applications using CM have been approved.1, 2 Although these approvals span small molecule drug product, small molecule active pharmaceutical ingredient (API), and biomanufacturing processes, the majority of the approvals are related to continuous manufacturing of small molecule drug products. Because this area of continuous manufacturing is the most mature within the pharmaceutical industry, this article focuses on some advantages and strategies that companies employing continuous drug product manufacturing have used to help accelerate development of solid oral products.
Continuous Direct Compression
The three primary manufacturing platforms (direct compression/encapsulation, dry granulation, and wet granulation) used for batch manufacturing of solid oral dosage forms are also used in continuous manufacturing. Analysis of 435 regulatory filings between 1996 and 2017 revealed that wet granulation is the most common manufacturing platform, with 38% of products using that process, followed by direct compression at 18% and dry granulation at 12%.3 Because the first application of a continuously manufactured drug product was not approved until 2015, these data overwhelmingly represent the manufacturing plat-forms that were selected for batch processes. However, a comparison of manufacturing platforms used for products publicly known to be made with continuous manufacturing shows a much different distribution: direct compression is the most common at 50%, followed by dry granulation at 33%, and wet granulation at 17% (see Table 1).4, 5, 6, 7, 8, 9 Although the pharmaceutical industry is still early in its journey with continuous manufacturing, it is interesting to note that early adopters of the technology tend to favor direct compression over the two primary granulation platforms.
Product | Company | Manufacturing Process | Reference |
---|---|---|---|
Orkambi | Vertex | Continuous wet granulation | 4 |
Prezista | Janssen | Continuous direct compression | 5 |
Verzenio | Eli Lilly | Continuous direct compression | 6 |
Daurismo | Pfizer | Continuous direct compression | 7 |
Symdeko | Vertex | Continuous dry granulation | 8 |
Trikafta | Vertex | Continuous dry granulation | 9 |
Determining which manufacturing platform to select for the development of a new molecular entity usually starts with a risk assessment or decision tree based on the unique requirements for the molecule being developed. Examples of items typically included in the risk assessment are (a) considerations related to the highest or lowest expected dose, (b) API powder physical properties (e.g., flowability, cohesiveness, compressibility), (c) API moisture sensitivity, and (d) API segregation potential. For example, the two primary reasons pharmaceutical engineers often select granulation platforms for batch processes are to minimize segregation potential and to improve the flowability of the blend due to high API concentrations in the formulation. However, when developing these risk assessments, it is important to note that the risk profiles may be quite different between batch and continuous processes due to the differences in the equipment operating principles. To illustrate, studies performed at Eli Lilly compared critical quality attributes (CQAs) of identical formulations, which were manufactured using both batch and continuous processes.10 The results demonstrated that the continuous direct compression process had equivalent or superior performance compared to the batch granulation process, and that all CQAs were achieved using continuous direct compression. This would suggest that continuous direct compression can be used on a larger percentage of assets compared to batch direct compression, thus enabling a platform-based approach to solid oral drug product development. The efficiencies of platform-based approaches are further discussed later in this article.
Formulation development for a direct compression process is generally simpler than for granulation processes because direct compression formulations usually have fewer ingredients (see Table 2), which leads to fewer formulation ranging and robustness studies. Moreover, direct compression processes help enable laboratory-scale formulation development because direct compression processes do not alter a material’s inherent compactibility properties as granulation processes can. As a result, prediction of how a formulation will compact on a continuous direct compression line based on batch laboratory data is much simpler than the analogous prediction for granulation processes.
The key information needed to successfully bridge laboratory-scale data with continuous data is an understanding of how lubrication in a laboratory-scale batch process translates to lubrication on the continuous line. Fortunately, models have been developed that are useful for predicting tensile strength changes as a function of lubrication for changes in scale of batch manufacturing processes.11 Models such as these can be modified and adapted to understand the relationship between lubrication at laboratory scale (for example, at a 50-gram scale in a Turbula mixer) and lubrication in the continuous process. The ability to perform formulation development at laboratory scale enables faster development timelines and reduced API consumption.
Direct Compression |
Dry Granulation |
Wet Granulation |
---|---|---|
|
|
|
* The solvent in wet granulation processes is removed, but it still must be studied and optimized during formulation/process development studies.
Finally, a continuous direct compression process in its simplest form only consists of three unit operations (loss-in-weight feeding, continuous mixing, and tablet compression), whereas granulation processes typically have at least three additional unit operations (e.g., roller compaction, milling, and blending of extragranular excipients). Not only does the simplicity of the direct compression process result in fewer process optimization studies due to fewer unit operations, it also leads to simplified control strategies, modeling, and material traceability/genealogy. Collectively, these advantages of continuous direct compression allow for more rapid formulation optimization, reduced process optimization studies, and accelerated development timelines.
Leveraging Platform Knowledge
The robustness of continuous processes described previously and the integrated nature of the unit operations allow practitioners of the technology to take a platform-based approach to the development of their solid oral portfolio. One of the greatest advantages to using a common platform across the portfolio is the ability to apply learning from one project to the next to increase development efficiency and accelerate timelines. Because continuous feeding and mixing are the two operations that are most different from batch processes, this section discusses approaches that have been used across continuous projects to streamline development.
Feeding
The first step in any drug product continuous manufacturing process is to feed the API and excipients into the process at the desired mass flow rates. The excipients used in continuous processes for solid oral products are generally the same as those used in batch processes, and a subset of excipients (e.g., microcrystalline cellulose, magnesium stearate, lactose, starch, and croscarmellose) tend to be commonly used across formulations.12 Because the feeding operation is ubiquitous across continuous lines and many of the excipients are common across formulations, databases containing information on feeding performance have significant potential to minimize experimental work from one project to the next. For example, once feeding performance is established for a given material and equipment setup, that knowledge can be used on any subsequent projects that use the same material and equipment setup.13. Moving beyond feeder performance databases, Yadav and colleagues14 and Wang et al.15 have combined feeding and raw material databases to build multivariate models that are used to predict feeding performance for drug product continuous manufacturing processes. These predictive models are particularly attractive during early process development not only because they minimize experimental work, but also because API availability is often limited at this stage of development.
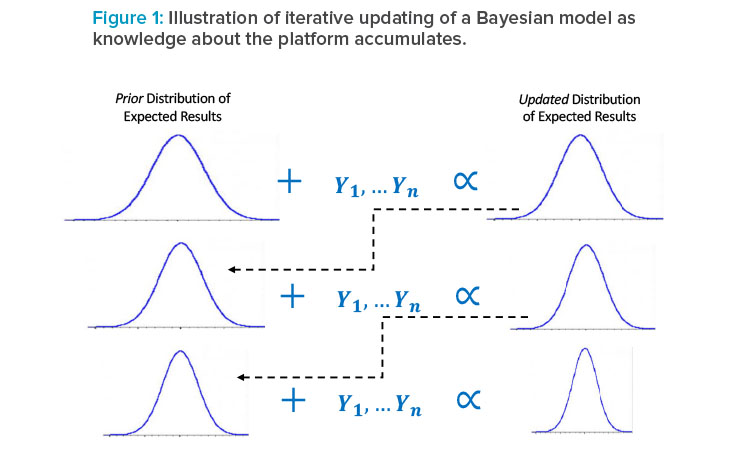
Mixing
In addition to using knowledge from the feeding operation to streamline development, drug manufacturers have also successfully used mixing studies performed on one asset to inform risk assessments and simplify development for subsequent assets. Roth and colleagues evaluated the impact of mixer paddle orientation, total mass flow rate, and mixer speed on the CQAs of a difficult-to-mix formulation at relatively low drug load (6.25%).16 The only parameter that had a meaningful impact on CQAs was the total mass flow rate. The authors used this information to fix the mixer paddle orientation and mixing speed within the continuous line for all subsequent assets, thus simplifying and streamlining development.
On the same continuous line, work performed by Manley and Shi demonstrated that large step changes in concentrations of microcrystalline cellulose, lactose, and mannitol result in similar response curves.17 Because the same equipment setup was used across these studies and only the formulations were changed, this finding suggests that the dispersion that occurs within a process across multiple formulations is comparable.17 This knowledge can be used when developing work plans for a new asset to ensure that the amount of mixing and dispersion is commensurate with the level of quality risk for the asset. Moreover, this finding could provide development organizations with a flexible, drug-substance-sparing option to characterize process dynamics.
Statistical Model
A natural component of a platform approach would be the use of Bayesian statistical methods, which can explicitly combine prior knowledge with newly observed data to obtain more precise models of expected performance than could be obtained with the newly observed data alone (see Figure 1).
Each bell curve represents a distribution of plausible results (e.g., values of a release or in-process test): previous knowledge about the expected results is characterized by a “prior” distribution (left column), which is combined in the Bayesian model with new data (e.g., from characterization or de-sign of experiments [DOE] runs of a new asset), resulting in an updated distribution of plausible results (right column), which then becomes the prior distribution for further experiments on the same or a subsequent asset. The width of the distribution reflects variability and statistical uncertainty, the latter being reduced in each update of the model as knowledge accumulates.
As represented in Figure 1, the model is iteratively updated as new knowledge about the platform is generated, resulting in an increasingly precise understanding of expected results with less new data needed than would be if this prior information were not leveraged in the modeling. This means that patients and manufacturers can benefit from reduced development costs and time without sacrificing understanding of process performance. For example, this prior information could be derived from DOEs performed in the development of previous assets or from validation or commercial runs of existing products. As with any use of information external to the asset currently being studied, the selection of prior information for a Bayesian model must be made carefully, ensuring that it is representative; once the representative prior knowledge is selected, the Bayesian approach provides a rigorous framework for using it, which mirrors the scientific process of continually updating knowledge.18
For example, the knowledge gained about the impact of process parameters and/or concentrations of formulation components on mixing and dispersion in the examples described previously could be explicitly and rigorously incorporated into models for new assets, reducing the amount of additional experimentation required to estimate performance and provide assurance of quality. Although still a less well-known approach in pharmaceutical process development, the use of prior information in Bayesian models has been used in adaptive clinical trial designs for some time.19 The approach is especially well suited to using a platform approach in the context of accelerated approval pathways, where the knowledge from previous assets on the same platform is “borrowed” to increase the precision of performance models with the new asset while using less new data than would be needed without leveraging this information.
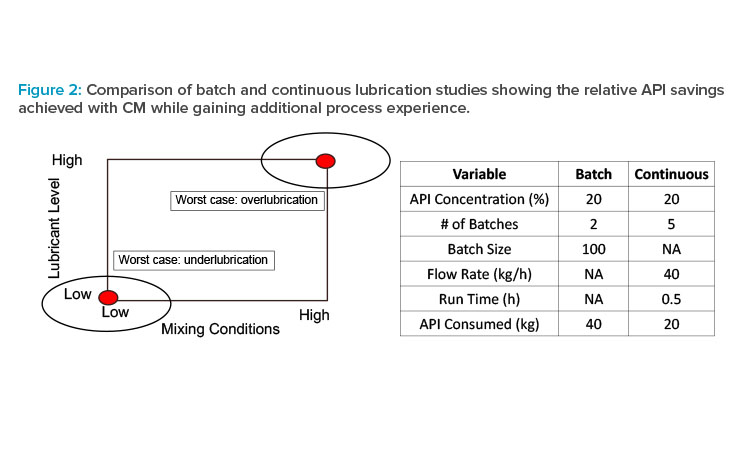
Bayesian modeling has other important advantages for continuous processes, regardless of whether prior information is incorporated into the model; in fact, in some scenarios, a Bayesian model is the most suitable approach to evaluate process performance and risk due to the complex data structures that often arise from continuous processes. Continuous manufacturing processes often generate a large amount of data, for example, from frequent process analytical technology (PAT) measurements incorporated into the control strategy (and sometimes as part of a real-time release testing strategy). In addition, validation of PAT measurement systems may involve confirmation of the comparability of PAT results to those from a traditional offline test method, such as high-performance liquid chromatography.
The resulting data structure is often complex, with multiple sources of variability represented within a single run (e.g., multiple measurements from each of multiple portions of a run [time intervals or in-process bins/hoppers]). Bayesian modeling is the optimal choice of statistical method in such cases to provide estimates of expected process variability (e.g., tolerance intervals), or the magnitude of expected bias between PAT and an offline measurement system for individual unit measurements, while accounting for these typical sources of process variability. Alternatively, Bayesian modeling can be used to directly predict the probability of meeting a specific release requirement related to the mean or variability within a batch (e.g., content uniformity), or to evaluate the probability of meeting requirements of a staged compendial test, such as dissolution, while still accounting for all sources of process variability. This approach was used by Roth and colleagues to evaluate the probability of meeting content uniformity requirements considering between- and within-location variability within a run, 16 and these features of Bayesian modeling are discussed in detail by Scherder and Giacoletti.20
Technical Transfer
Perhaps the most widely publicized benefits of continuous manufacturing are related to the ability to perform development studies at a commercial scale while minimizing the amount of API consumed in the study. With traditional batch manufacturing, the batch size is generally dictated by the equipment size available to process the material. Therefore, running commercial-scale experiments as part of development work plans would require significant amounts of API and is not feasible in most situations. However, with continuous manufacturing, commercial-scale equipment is used in development without consuming significant amounts of API because the process can be run long enough to collect the required data to ensure process robustness and then the experiment can be stopped.
As an illustrative example of this approach, consider lubrication blending of powder mixtures just prior to final dosage-form formation. Powder lubrication is essential to facilitate processing (e.g., preventing picking/sticking and minimizing ejection stresses during tableting), but over-or underlubrication can have a significant impact on CQAs such as dissolution or appearance. Powder lubrication in scale-up of batch processes has historically been poorly understood, and though scale-up models exist,11 they are empirical in nature. As a result, blending studies with batch processes are frequently performed at or near commercial scale prior to pivotal clinical studies to minimize risk of formulation changes after the pivotal studies have started. Parameters impacting these blending studies are often grouped together to represent worst-case scenarios for both under- and overlubrication (see Figure 2); in other words, the amount of API needed for full-scale studies is inefficient not only from a materials point of view but also from an experimental design perspective.
For illustration, consider a relatively modest batch process batch size of 100 kg with 20% drug load. A worst-case approach consists of two batches representing the extreme lubrication conditions, which provides information only at those conditions (i.e., one cannot mathematically interpolate to other combinations) and requires 40 kg of API. By contrast, a DOE can be implemented easily and efficiently using a continuous process, generating much more process knowledge using half the amount of API. For example, with a total mass flow rate of 40 kg/h and a run time of 30 minutes, a two-factor, two-level factorial DOE with a single center point (five runs) uses 20 kg of API but generates far more knowledge, which increases understanding and decreases risk. The additional process understanding (e.g., impact on mixing of conditions other than the worst case and at combinations not actually run but within the experimental space, detection of interactions is not only valuable for the present process but also can be leveraged for future assets as part of a platform approach. Collectively, this approach can be used to accelerate development because it reduces technical risk for the current asset and becomes prior knowledge for future assets while at the same time reducing API consumption.
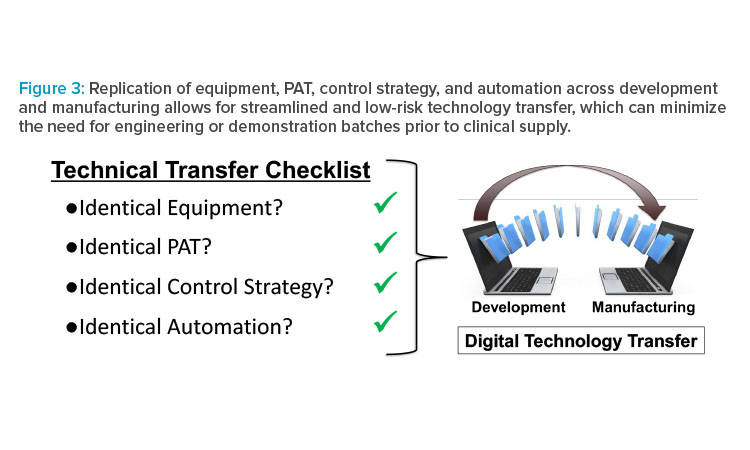
As a result of being able to perform development studies in commercial-scale equipment, continuous manufacturing offers a unique opportunity to exactly replicate equipment sets across development and manufacturing. This approach has been implemented by companies21,22 and can result in significantly reduced technical risk, increased development efficiency, and accelerated time to reach the clinic. By performing development studies using the same equipment, PAT, control strategy, and automation, the benefits of running an engineering or demonstration batch at the manufacturing site prior to making clinical material are significantly diminished. By eliminating demonstration batches, technology transfer can be accomplished via a digital transfer of the automated control strategy and enable an organization to move directly into GMP clinical supply (Figure 3). This paradigm may be particularly advantageous for new molecular entities, which are targeted toward life-threatening conditions that may qualify for some type of expedited regulatory review. With approximately 25% of all new drug approvals in the US receiving the FDA’s breakthrough designation,23 anything that chemistry, manufacturing, and controls organizations can do to accelerate medicine to the clinic is a win for patients.
Flowsheet Models as Digital Risk Assessments
Continuous processes naturally lend themselves to flowsheet modeling due to the integrated nature of the unit operations that comprise them. Flow-sheet models can serve many purposes, including facilitation of process design, process optimization, and material traceability and genealogy.24 But when considering accelerated development scenarios, perhaps the greatest value that flowsheet models offer is the ability to leverage them as a way to perform digital risk assessments for the process and product being developed. When used in this manner, flowsheet models allow more targeted and focused experimentation on those elements of the process that may present higher risk to product quality.
An example of how flowsheet models can be applied to accelerate development is to consider one of the primary differences between batch manufacturing and continuous manufacturing: how the input materials are charged into the system and mixed. With batch processes, all materials to be mixed together are charged into the processing equipment at once. Mixing robustness studies and validation of the batch mixing process ensure acceptable uniformity of the material at the appropriate scale of scrutiny. In this regard, the homogeneity of the material in a batch process is assumed to be independent of time once blending is complete.
However, with continuous processes, materials are fed into the system at desired mass flow targets for a defined period of time. As such, the actual mass flow rates of the input material have the ability to vary over time-based on material properties, natural process variability, and equipment performance. Flowsheet models can be used to understand the impact that this mass flow variability can have on the composition of the blended material prior to final dosage form formation (i.e., tablet compression or capsule filling). For example, García-Muñoz and colleagues built a flowsheet model that was used to predict the impact that a loss-in-weight feeder mass flow disturbance would have on the API concentration at the tablet press feed frame.25 By using the model to simulate a range of feeder disturbances, contour plots were generated that depict the region where a disturbance is dampened within assumed limits.25 Models such as these are invaluable tools for reducing experimental burden because they can be used as a digital risk assessment to focus the experimental plan and set limits as part of the control strategy.
Conclusion
In the increasingly competitive environment of the pharmaceutical industry, speed to the clinic and market has never been more important, not only for patients who are waiting for medicines but also to maintain business viability. As pharmaceutical scientists and engineers, we are well positioned to leverage technology to accelerate development of the manufacturing processes used to supply medicine to the clinic. One such technology that can be used for accelerated development is continuous manufacturing, which is still in its early phase of implementation within the pharmaceutical industry.
Continuous manufacturing offers multiple advantages related to reducing development timelines. As illustrated by the publicly known approvals of drug products made using CM, it appears that direct compression may play a larger role than other platforms commonly used for batch processes (e.g., dry or wet granulation). With fewer unit operations and generally simpler formulations than would be needed for a batch process for the same product, continuous direct compression allows for fewer formulation and process optimization studies, which in turn enables speed to market. In addition, continuous processes can save significant quantities of API during development for multiple reasons, including no scale-up, the ability to leverage continuous processes more consistently as platforms, and the integration of continuous processes with modeling that can be used to reduce and focus experimental plans. By saving API in drug product development, API manufacturing campaigns could potentially be reduced in size, resulting in faster deliveries and speed to the clinic.