Data Science for Pharma 4.0™: Drug Development, & Production—Part 2

This second of a two-part series explores digital transformation and digitalization in the biopharmaceutical industry with information about how data science enables digitalization along the product life cycle. (Part 1 was published in the March-April 2021 issue of Pharmaceutical Engineering.1 )
- 1Herwig, C., et al. “Data Science for Pharma 4.0™, Drug Development, and Production—Part 1.” Pharmaceutical Engineering 41, no. 2 (2021): 40–47.
In the biopharmaceutical industry, the entire product life cycle—from the fundamentals of medicine and biological science to research and development, to manufacturing science and bioprocessing validation—is being transformed and even disrupted through the capabilities of data science, digitalization, and the industrial internet of things (IIoT), all of which fall under the umbrella of Industry 4.0. Data-driven innovations can improve the flexibility of production by enabling rapid adjustments of scale and output to reflect sales forecasts, and they allow quick adaptations of product ranges to new market demands based on a profound knowledge of the manufacturing platform.
However, Industry 4.0 features can only succeed in the biopharmaceutical industry if they build upon and improve established concepts. For example, Industry 4.0 should advance defined procedures (such as batch reviews) and corrective and preventive actions (CAPA) management following ICH Q10 principles,2 and it should support the introduction of novel life-cycle management concepts, such as those exemplified in ICH Q12.3
In this article, we analyze how data science enables digitalization along the product life cycle. We start with technology transfer, as Part 1 of this series already addressed the general tools relevant to the process development stage. We discuss data science tools relevant to manufacturing and its augmented periphery, such as logistics and the supply chain, as well as new modalities such as advanced therapy medicinal products (ATMPs). Finally, we discuss what is needed to ensure the industry is ready to use these tools effectively, provided they are available.
Figure 1 illustrates how Industry 4.0 enablers, including digitalization, affect the product life cycle, starting with the establishment of a quality target product profile (QTPP) in drug discovery and development. Figure 2 highlights the uses of data science tools throughout the life cycle.
Technology Transfer and Process Validation
A shift of emphasis from flexibility to increased control is inevitable as the development of biopharmaceutical products matures via technology transfer from process development to process validation. Statistical software for design of experiments and multivariate analysis is widely used in the transitions from process development through process characterization to process validation. However, software use is hindered by format incompatibility issues, the complexity of the data sets, responsibility questions, organizational boundaries, and other challenges. Consequently, early research and development data and technology transfer data may not be captured, processed, and stored in a structured manner that would allow them to be used to their full potential.
ICH Q12 outlines how product quality assurance is achieved through established conditions (ECs) for manufacturing and control, which are legally binding information in regulatory applications.3 Companies should provide supportive information for ECs in regulatory documentation to justify their selection. In our experience, the biopharmaceutical industry lacks the establishment of an initial design space in practice and its maintenance throughout the life cycle as part of continuous improvement. Provided data integrity is in place, data science can create a holistic production control strategy2 ,3 ,4 ,5 ,6 ,7 for technology transfer and process validation, and this strategy could be a key enabler for generating information to support selected ECs in regulatory submissions.
- 2 a b International Council for Harmonisation of Technical Requirements for Pharmaceuticals for Human Use. “ICH Guideline Q10 on Pharmaceutical Quality System.” European Medicines Agency website. Published 2008. https://www.ema.europa.eu/en/documents/scientific-guideline/international-conference-harmonisation-technical-requirements-registration-pharmaceuticals-human_en.pdf
- 3 a b c International Council for Harmonisation of Technical Requirements for Pharmaceuticals for Human Use. “ICH Guideline Q12 on Technical And Regulatory Considerations for Pharmaceutical Product Lifecycle Management Step 5.” European Medicines Agency website. Adopted January 2020. "https://www.ema.europa.eu/en/documents/scientific-guideline/ich-guideline-q12-technical-regulatory-considerations-pharmaceutical-product-lifecycle-management_en.pdf
- 4Paul-Ehrlich-Institut, Federal Institute for Vaccines and Biomedicines. “Advanced Therapy Medicinal Products (ATMP).” 2019. https://www.pei.de/EN/medicinal-products/atmp/atmp-node.html
- 5Herwig, C., C. Wölbeling, and T. Zimmer. “A Holistic Approach to Production Control.” Pharmaceutical Engineering 37, no. 3 (2017): 44–49.
- 6International Society for Pharmaceutical Engineering. ISPE Baseline Guide, Vol. 5, Commissioning and Qualification, 2nd ed. North Bethesda, MD: International Society for Pharmaceutical Engineering, 2019.
- 7International Society for Pharmaceutical Engineering. GAMP® 5 Guide: Compliant GxP Computerized Systems. North Bethesda, MD: International Society for Pharmaceutical Engineering, 2008.
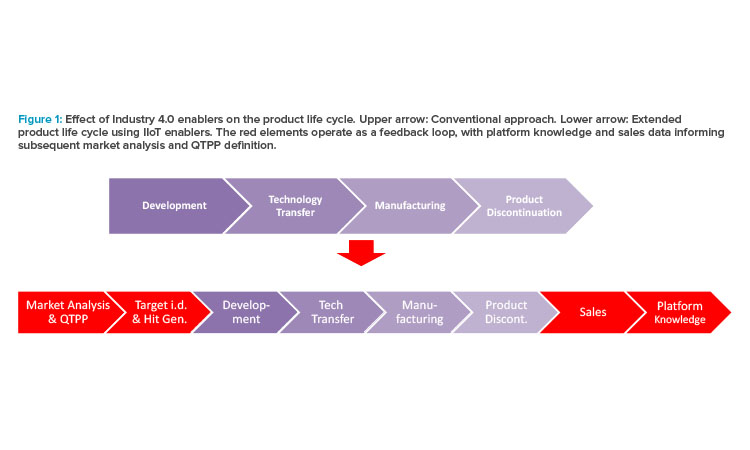
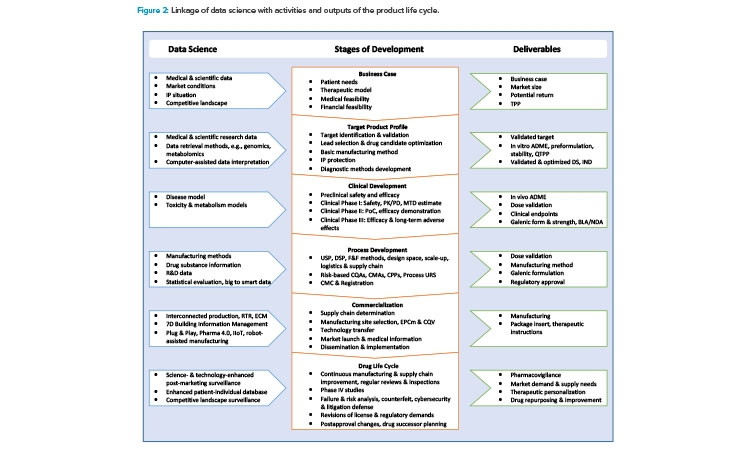
In the bioprocessing industry, the appropriate data science methods to generate supportive information for ECs are highly dependent on the product type. Although the bioprocessing industry is generally considered a novel segment of the pharmaceutical industry, there is considerable diversity in the degrees of maturity and complexity among different bioprocesses. This diversity strongly affects how one should implement data science.
Monoclonal antibody (mAb) manufacturing represents, by far, the main bioprocessing class, and it is characterized by a sequence of very similar and relatively well-known process steps. These processes are usually placed into a platform process, which is applicable for different mAb products. This enables manufacturers of mAb products to apply the same knowledge to different products, thus shortening development timelines by, for example:
- Prealigning the sending and receiving units for smooth technology transfers
- Using established equipment scalability
- Establishing platform knowledge to support the control strategy
- Ensuring consistency in the starting materials used throughout the product life cycle
A data science implementation strategy to support these relatively standardized bioprocesses may be empowered using generic tools as the starting points. Those tools include:
- Automated data import via real-time interfaces, API configurations, and file crawlers, as well as linking data lakes with laboratory information management systems (LIMSs), historians, and electronic laboratory notebooks (ELNs)
- Data contextualization between the various data sources and data types, such as aligning time-value pairs with discrete product quality measurement and with features extracted from morphological images
Using these types of data science tools for mAbs allows an immediate return on investment (ROI) in technology transfer and process qualification tasks by supporting risk assessments and mitigations. Also, in the long term, such tools support biological license application (BLA) submissions and continued process verification (CPV) in commercial production. Note that any generic tool will need some level of customization because process and analytical data are currently automatically captured in a variety of formats and often need to be cleaned from noise.
ATMPs represent another class of products within the bioprocessing industry. Recently, an increasing number of ATMPs have received market approval; however, some have subsequently been taken off the market [4]. Unlike mAbs, ATMPs are manufactured by “nonstandard” processes with a high degree of complexity characterized by the following:
- Varying equipment and procedures, even for the same product category
- Limited prior knowledge to ensure smooth technology transfer
- Unavailability of some raw materials and reagents in large-scale amounts or at GMP grade
Data science implementation for ATMPs probably cannot leverage prior knowledge to the extent achieved with mAbs. Customized tools may therefore be required from the start. The short-term ROI from data science implementation in ATMP development may be more limited than in the more standardized mAb manufacturing process. However, the mid-to long-term ROI of data science for ATMPs may be considerable. By capturing all available process and analytical data for ATMPs, data science tools may help investigators detect critical correlations that would not become apparent from conventional data analysis. Thus, data science is likely an enabler for ATMP manufacturing, not despite the complexity of these products, but because of it.
Technology transfers often involve multiple organizations or companies (e.g., contract manufacturing organizations), a range of operational procedures, and even multiple languages. Data science integration may therefore be complex even for well-known, almost commodity, bioprocessing products, such as mAbs. This complexity may be one of the main obstacles in the implementation of a comprehensive data science strategy. Another implementation challenge may be the lack of a dedicated data science representative on the technology transfer team.
A quality agreement between the sending and receiving units defining the responsibilities and operational modes of data sharing is an important priority. Many important relationships between process inputs and outputs have already been established for mAbs. Therefore, data science innovations may be less urgently needed for successful technology transfer and process validation in mAb manufacturing than in the ATMP sector.
Life-Cycle Considerations
Following process validation, manufacturing operations must demonstrate process compliance within established proven acceptable ranges, following isolated process parameter control strategies as well as holistic production control strategies.5 Demonstrating compliance requires that the ECs be clearly identified as per ICH Q12. Companies therefore need to:
- Demonstrate that the product is continuously within its specifications through CPV;
- Predict that the product will meet specifications through real-time release testing;
- Perform trend analysis and identify batch-to-batch variability, which may occur due to variations in raw material, human interactions, or equipment ag-ing, and set appropriate actions for preventive maintenance and CAPA (data science is an acknowledged tool for root-cause analysis);
- Manage postcommercialization data regarding sales, regulatory changes, published data, change management; and
- Provide the assembled process knowledge and wisdom obtained for the product in a comprehensible format to the company’s product pipeline.
Production Control Strategies
Biopharmaceutical manufacturers need the data gained during process development to be reduced and integrated into a manufacturing environment at production scale. Because this transformation process is highly demanding for biopharmaceutical drugs due to the complexity of process parameters and product characteristics, it should be anticipated from early development. The data science concepts and tools described in Part 1 of this series and the previous sections of this article not only help stakeholders characterize the manufacturing process, specify the relevant critical quality attributes and critical process parameters, and prepare an application dossier and a master batch record, but also are required to design a verifiable, robust, reproducible, and highly intensified manufacturing strategy that will pay off quickly. The current data reduction processes can miss some key information in the technology transfer to commercial production. A more holistic approach to production control based on process and data maps from development to production should help improve the reliability and robustness of the production process greatly. The necessary tools are available and need a regulatory framework for implementation.5
Facility Design
When it comes to designing a production plant, the quality of process simulation for concept validation requires special attention. Results strongly depend on the quality of the underlying data and the simulation model setup; experienced specialists are needed for this work. Data quality must address errors, outlier detection, and, above all, data integrity.
Currently available models depend on extensive data reduction and cannot completely mitigate scale-up risks. A recent and increasingly popular alternative is the scale-out approach, which avoids the scale-up and thereby provides data from late development directly to the market production scale, and vice versa. Starting from a concise process description, a suitable manufacturing environment needs to be designed, accessory processes and systems identified, and the scope and interfaces defined. Plant engineering, construction, and life-cycle management become increasingly reinforced by integrative data platforms with the ability to take in all necessary information from various sources and provide a real-time overview of any aspect of the plant at the push of a button. Highly desirable for engineering and life-cycle plant management are standardized and interchangeable formats being fostered in the Data Exchange in the Process Industry (DEXPI) project. Currently, DEXPI mainly focuses on a common format for the exchange of piping and instrumentation diagrams, and, as mentioned in Part 1 of this series, it is of increasing interest to stakeholders in the process engineering industry.
A good facility design provides for optimal manufacturing conditions based on the information gained in process development. GxP compliance further needs to be ensured throughout the main supply, support, manufacturing, release, and distribution chain. The amount of data generated to guarantee reliability and compliance in manufacturing is huge, and the data require continuous reduction, evaluation, and correlation to maintain safe production and support continuous improvement. Data from several systems are considered to be highly critical,6 and in the United States they require initial validation and compliance with Title 21 CFR Part 11. Automation concepts currently rely on manual configuration of data transfer protocols and are static by design. Therefore, they cannot meet the current needs for flexibility in process flow and scale, support a unified data storage and exchange method, or help with a swift integration of improved processing methods into the manufacturing environment. Here, plug-and-produce concepts are beneficial alternatives: They offer standardized formats for data exchange between the lower and higher functional levels, and they promise both the seamless integration of equipment and systems and the integrity of data by design. However, some systems in the automation landscape (namely, building management, enterprise resource planning, and document management systems) are designed for general purposes, and will continually need manual adaptation for use in a pharmaceutical production environment.
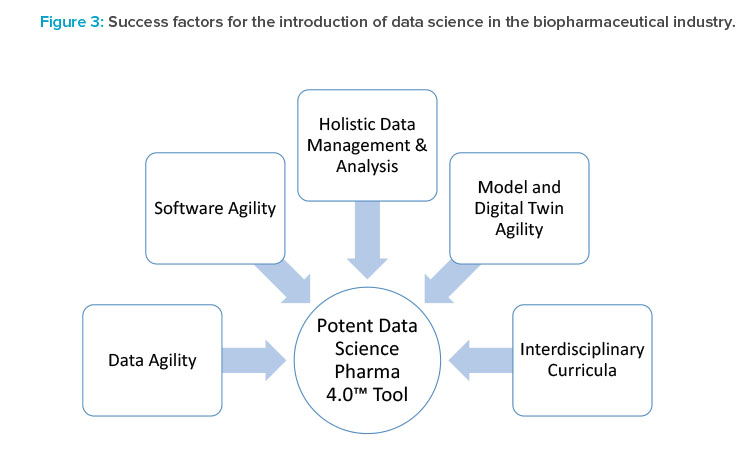
ATMP Manufacturing and Logistics
When a chimeric antigen receptor T cell (CAR-T) therapy needs patient cells as a starting point for drug manufacturing, the process of blood sampling and cell preparation at the hospital has to be documented in a GxP-compliant manner, the integrity of both the transport conditions and the patient’s information needs to be ensured, and all those data finally have to be included in the product’s batch record. This is a real paradigm shift: ATMP manufacturing must not only attend to product safety and quality aspects, but also protect the integrity and confidentiality of patient information. The procedures established for organ transplants across extended distances and relying on a central database and assisted transport may illustrate the complexity of such concepts, but those procedures are far too expensive for application in ATMP therapies. Given the pressures, ATMP production calls for holistic data acquisition along the therapeutic and manufacturing chain; this approach is already partially applied to the compounding of cytostatic drugs.
Some gene therapeutic concepts have even deeper connections to data science. The possibility of producing an RNA vaccine based on the gene expression profile of a patient’s tumor directly links patient information to drug manufacturing. In the manufacture and distribution of this type of product, the patient’s identity and clinical data that may be of interest to third parties must be protected. An integrated data safety model consisting of different layers of protection for data safety and integrity for all aspects should be implemented. Starting with a system that is validated according to GAMP® 57 for all critical parts of the diagnostic and manufacturing chain, data integrity must be ensured by the system operations that control the validated state. A third layer of data integrity should be set up for the complete IT management system involved, including, for example, hosted services. Finally, a concept should be in place to ensure cybersecurity throughout the data chain. For these reasons, digitalization and data integrity shall be seen as requirements for data science.
Another consideration is that the drug manufacturer could possibly use a scientific data model to intervene directly in the therapeutic strategy chosen for a patient. Data science may thus not only enable progress in drug manufacturing, but also enhance therapeutic success. Detailed therapeutic data may further return from the patient’s hospital to the drug license owner and improve the data model, with implications for both the therapeutic strategy and the manufacturing process.
Holistic Data Science Concept
Industry 4.0 involves the integration of many individual tools. Some data science tools already exist and are in use. However, the individual data science tools have no Pharma 4.0™ relevance unless they are integrated into a holistic data science concept.
- Several levels of integration are needed to turn individual data science tools into a powerful Pharma 4.0™ environment (Figure 3). Integration of such tools needs to be flexible by design because the outcome should be a flexible manufacturing platform for multiple products and include a product life-cycle approach. The following are key priorities:
- Software agility: We propose DevOps techniques and a DevOps mindset, as an agile approach, without gigantic deployment, test, or validation overheads. Although DevOps applications are currently successful, especially for software-as-a-service (SaaS) deployment, DevOps is not yet fully established for IT/operational technology (OT) environments in pharmaceutical companies (see Part 1 of this series).
- Data agility: SaaS cloud solutions will be the future basis for data and knowledge exchange, although cybersecurity concerns must first be solved technically and at the political level. Moreover, we are convinced that SaaS tools will provide the agility required for exchanging data as well as model and digital twin life-cycle management.
- Holistic data management and data analysis: Plug-and-produce solutions, as exemplified in the Pharma 4.0™ Special Interest Group (SIG) initiatives, include standardized data interfaces and consistent data models. Data availability, however, is not sufficient. We also need the ability to holistically analyze different data sources and integrate time-value data sets, spectra, images, ELNs, LIMSs, and manufacturing execution system data—these are being targeted in the Pharma 4.0™ SIG Process (Data) Maps, Critical Thinking workgroup.
- Model and digital twin agility: To ensure that data models and digital twins can be adapted along the life cycle and continuously deployed in a GxP environment, the industry needs a flexible, but still validatable, environment for capturing and deploying knowledge. This environment may include artificial intelligence and machine learning, as well as hybrid solutions developed for all kinds of scenarios. Therefore, we need validated workflows for automated model development and digital twin deployment, including integrated model maintenance, model management, and fault detection algorithms.8 ,9 This should be an extension of the GAMP® 5 guidance.7
- Interdisciplinary teams: Numerous data scientists will be required to run the facilities of the future. Standardization of workforce development should help ensure that expectations for training and proficiency are uniform across the industry. Agreement on standard curricula and assessment measures would facilitate this. Initiatives should be launched at universities and governmental organizations but should also involve industry training centers.
Conclusion
Digitalization in the bioprocessing industry is advanced by focusing on knowledge and integrating the complete spectrum of data science applications into the product life cycle. The industry needs life-cycle solutions, such as feedback loops in CPV, for knowledge management. The data science framework for these solutions is already set, but we need to set up business process workflows according to ICH Q10 guidance, automate PCS workflows, and agree on core plots for trending of regular manufacturing and CPV solutions. We have to “live” ICH Q12, facilitated by data science tools.
The main obstacle to achieving this goal is convincing all industry stakeholders—individuals, teams, groups, departments, business units, management, leadership, and C-level executives—of the benefits of making value out of data. Google and Facebook may serve here as well-known examples of companies that have translated data into value.
The industry urgently needs to invest in interdisciplinary curricula as a midterm strategy. And those of us who are data science–trained engineers are obligated to show the benefits of integrated tools and workflows and explain what the industry essentially needs throughout the product life cycle.
Data Science for Pharma 4.0™, Drug Development, & Production—Part 1
Digital transformation and digitalization are on the agenda for all organizations in the biopharmaceutical industry.
- 7 a b
- 8US Food and Drug Administration. “Proposed Regulatory Framework for Modifications to Artificial Intelligence/Machine Learning (AI/ML)-Based Software as a Medical Device (SaMD)—Discussion Paper and Request for Feedback.” 2 April 2019. https://www.regulations.gov/document?D=FDA-2019-N-1185-0001
- 9Pathmanathan, P., R. A. Gray, V. J. Romero, and T. M. Morrison. “Applicability Analysis of Validation Evidence for Biomedical Computational Models.” Journal of Verification, Validation, and Uncertainty Quantification 2, no. 2 (2017): 021005. doi:10.1115/1.4037671